the Creative Commons Attribution 4.0 License.
the Creative Commons Attribution 4.0 License.
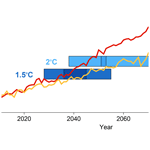
Approaches to attribution of extreme temperature and precipitation events using multi-model and single-member ensembles of general circulation models
Sarah E. Perkins-Kirkpatrick
Andrew D. King
Extreme temperature and precipitation events occurring in Australia in recent decades have caused significant socio-economic and environmental impacts, and thus determining the factors contributing to these extremes is an active area of research. Many recently occurring record-breaking temperature and rainfall events have now been examined from an extreme event attribution (EEA) perspective. This paper describes a set of studies that have examined the causes of extreme climate events using various general circulation models (GCMs), presenting a comprehensive methodology for GCM-based attribution of extremes of temperature and precipitation observed on large spatial and temporal scales in Australia. First, we review how Coupled Model Intercomparison Project Phase 5 (CMIP5) models have been used to examine the changing odds of observed extremes. Second, we review how a large perturbed initial condition ensemble of a single climate model (CESM) has been used to quantitatively examine the changing characteristics of Australian heat extremes. For each approach, methodological details and applications are provided and limitations highlighted. The conclusions of this methodological review discuss the limitations and uncertainties associated with this approach and identify key unexplored applications of GCM-based attribution of extremes. Ideally, this information will be useful for the application of the described extreme event attribution approaches elsewhere.
- Article
(2604 KB) - Full-text XML
- BibTeX
- EndNote
1.1 Australian weather and climate extremes
Since around the year 2000, many high-impact extreme and record-breaking temperature and precipitation events have occurred over Australian regions (Bureau of Meteorology, 2013a, b, c, 2016a). During 2001–2010, the Murray–Darling Basin (MDB) region, in south-eastern Australia, experienced an extreme dry period that had severe environmental and socio-economic consequences (Van Dijk et al., 2013). The Millennium Drought in the MDB (defined here as the period from 2001 to 2010) was the longest uninterrupted series of years since at least 1900 with below median observed rainfall. The persistent heavy rainfall of 2010–2012 (Bureau of Meteorology, 2012) ended the drought of the preceding decade but resulted in severe flooding in northern and eastern Australia. This led to billions of dollars of flood damage, the evacuation of thousands and 33 deaths (Queensland Floods Commission of Inquiry, 2012). Furthermore, following the consecutive strong La Niña events of 2010 to 2012, a period of well above-average temperatures occurred across Australia (Bureau of Meteorology, 2014). In the period since, record-breaking extremes were observed on sub-daily to multi-year timescales and on spatial scales ranging from single stations to Australia-wide (Bureau of Meteorology, 2016b). Many of these events were the most extreme since high-quality national records began in 1910.
Australia has a highly variable climate that is influenced by several large-scale climate modes such as El Niño–Southern Oscillation (ENSO), the Interdecadal Pacific Oscillation (IPO), the Indian Ocean Dipole (IOD), the Southern Annular Mode (SAM), the Subtropical Ridge (STR), and the Madden Julian Oscillation (MJO) (King et al., 2014; Maher and Sherwood, 2014; Min et al., 2013). These large-scale modes may also interact with local- and regional-scale processes, such as soil moisture feedbacks, to impact the severity, duration or likelihood of extreme events such as heatwaves (Perkins et al., 2015). Anthropogenic climate change is also a key influence in the observed characteristics of some extreme weather and climate events (Herring et al., 2018). Understanding the influences of both these anthropogenic forcings and/or natural climatic variability on recent temperature and rainfall extremes affecting Australia, as well as elsewhere, has become an active research avenue.
1.2 Extreme event attribution
Extreme event attribution (EEA) studies focus on understanding a particular observed extreme weather or climate event. These studies typically combine observational and model data to determine whether various factors (e.g. anthropogenic greenhouse gas composition changes) contributed to a specific observed aspect of an observed extreme event, such as its intensity, magnitude or frequency. Likelihood-based EEA approaches compare the probability of occurrence of an extreme event in the current climate with the occurrence in a counterfactual climate without anthropogenic climate change. There are a number of different methods possible for applying the risk-based EEA approach, including using observations, regional climate models, or coupled or atmosphere-only general circulation models (GCMs).
Numerous previous studies have made quantitative assessments of the influences of anthropogenic forcings on the likelihood of an observed event using fraction of attributable risk (FAR) values. A FAR value is usually defined as
where PNAT denotes the probability of an event occurring in a reference state and PALL under a parallel forced state (Stone and Allen, 2005). The probability of a defined event occurring may, for example, be calculated in a large model ensemble of climate models and then compared to the equivalent event probability in a parallel counterfactual model experiment, such as where only natural climate forcings (e.g. volcanic aerosols and solar irradiance) are imposed. The FAR value provides a quantification of the change in probability of the defined event occurring that can be attributed to a particular cause, specifically the difference between the model experiments (i.e. anthropogenic climate forcings). The FAR approach has been widely implemented in event attribution studies (see Herring et al., 2014, 2016, 2015), though the details of its calculation vary.
This paper contributes to a series that outlines different methodological approaches to examining and applying event attribution approaches to observed extremes. Many extreme weather and climate events occurring in Australia have now been examined from an event attribution perspective using a cognate methodology. Broadly, this methodology is a quantitative event attribution approach using GCMs and some Earth system models (ESMs), which is outlined in detail here. As a large number of studies have now been conducted focused on Australian weather and climate extremes (see Lewis et al., 2017b), we detail the methodological approaches used in these analyses. In this paper, the climate models and attribution approaches that have been applied to Australia's observed extremes are described and compared with a level of methodological detail not provided previously. The specific purpose of this contribution to this EEA-focused series is (i) to provide detailed information about model-based EEA studies applied to Australia and hence (ii) to provide guidance for others aiming to interpret these previously published results or ideally apply the described EEA approaches elsewhere.
The Australian-focused studies detailed in our paper use differing EEA model frameworks that we call either multi-model or single-model ensembles, with the applications, advantages and limitations of each discussed throughout. The multi-model ensemble is constituted by many different climate models, each with a small number of contributing ensemble members or realizations. The single-model ensemble is alternatively constituted by a large number of ensemble members of one model only that differ in terms of model physics or initial conditions in order to sample internal climate variability. Each model framework provides a different level of conditioning, which provides a methodological focus on specific aspects of weather and climate such as sea surface conditions. This degree of conditioning is important for interpreting attribution statements (National Academies of Sciences, Engineering, and Medicine, 2016) and is discussed throughout.
2.1 Multi-model ensemble (CMIP5) description
In the first examples, attribution statements on observed extremes were made by analysing models participating in the Coupled Model Intercomparison Project Phase 5 (CMIP5) (Taylor et al., 2012). CMIP5 provides a framework for coordinated and standardized climate change experiments, including detection and attribution experiments. More than 50 models, from over 20 international groups, participated in CMIP5 by contributing data, although the number of models and realizations varies depending on experiments. Experiments are tiered by importance and participation for each experiment is voluntary. All the models are fully coupled (atmosphere–ocean GCMs and ESMs) and of varying horizontal and vertical resolutions, and are run under standardized time-evolving forcings (e.g. time-varying concentrations of various atmospheric constituents such as greenhouse gases).
Multiple CMIP5 experiments can be used together to analyse changes in the characteristics of observed weather and climate extremes that are associated with various forcings. These experiments are
-
historical, 1850–2005, using time-evolving atmospheric compositions due to observed anthropogenic and volcanic influences, solar forcings and emissions of short-lived species from natural and anthropogenic aerosols.
-
historicalNat, 1850–2005, using time-evolving volcanic influences and solar forcings.
-
piControl. Control runs provided for each model which are long, freely evolving climate simulations with greenhouse gas concentrations appropriate for circa 1850 that permit the analysis of a large number of model years.
-
rcp8.5. Representative Concentration Pathway (RCP) simulations of 2006–2100. RCPs are scenarios that assume particular policies are implemented to achieve greenhouse gas emissions targets. These scenarios include a range of future projections for future populations and technological responses (Moss et al., 2010). For instance, in the rcp8.5 future scenario, the radiative forcing increases before reaching a level of about 8.5 W m−2 at the end of the century.
We note that the use of multiple CMIP5 models does not condition to aspects of the observed state of the climate (such as sea surface temperatures) that prevailed during the event in question. Simulated SSTs in a given year in CMIP5 realizations do not relate directly to those observed. This unconditional approach using CMIP5 is described as the most comprehensive and easy to interpret (National Academies of Sciences, Engineering, and Medicine, 2016, p. 51), as all aspects of weather and climate are considered and analysis is not limited to particular climatic situations. Coupled model ensembles may be used with a degree of conditioning on climate modes by subsetting climate model years characterized by a given mode of variability (e.g. the NINO3.4 index could be calculated in models to discriminate between phases of the El Niño–Southern Oscillation). This approach has been used in EEA studies, but the conditioning is still, by necessity, less restricted than for atmosphere-only simulations (discussed further in Sect. 2.3).
2.2 Single-model ensemble (CESM) description
We next describe a single-model ensemble for EEA, which samples the influence of internal variability in the attribution of climate extremes to human influence. In recent years, this approach has been demonstrated using two versions of the Community Earth System Model (CESM). The first version is a 21-member ensemble of CESM-CAM4, run at approximately 2∘ resolution (Fischer et al., 2013). The second, newer version is the 40-member CESM-LENS ensemble, run at 1∘ resolution (Kay et al., 2015). Of course, the methods and examples described below could (and should) be replicated for other climate models with similar resources (see descriptions in Kirchmeier-Young et al., 2017, for example). This would allow for an estimate of the influence of internal variability, combined with at least some structural differences across individual climate models, to be made systematically for events.
The specifics of the multi-member CESM ensemble differ slightly compared to CMIP5. Firstly, instead of a historicalNat simulation, a long control is used to represent the counterfactual world. Here, greenhouse gas forcings are held to pre-industrial levels, and the model freely evolves under these constraints for the length of the simulation. Secondly, all realizations forced by historical and anthropogenic emissions are identical, except in a tiny atmospheric perturbation in the atmospheric temperature initial conditions of each simulation. This is enough to trigger completely different realizations of internal variability over the length of the simulation. The start of each realization is forced by historical conditions, and the rcp8.5 scenario from the start of 2006. The older version of CESM employed in attribution studies has a control run of 982 years and 21 anthropogenically forced realizations that commence in 1950. This compares to the newer version with a control run of 1800 years and 35 anthropogenically forced realizations that commence in 1920. The CESM ensemble provides an unconditional attribution framing.
2.3 Other model ensembles
We refer later to CMIP5 comparisons with results that are also derived from other model frameworks, which we describe here. First, we describe the Attribution of extreme weather and Climate Events (ACE) framework (Stott et al., 2016). Unlike the coupled ocean–atmosphere CMIP5 experiments, these are atmosphere-only model simulations driven by prescribed sea surface temperatures (SSTs). This model approach uses two large ensembles of simulations over the extreme event period: one ensemble simulates the actual climate, including all known external forcings (anthropogenic forcings are long-lived greenhouse gases, aerosols, tropospheric and stratospheric ozone and land-use changes, and natural forcings are from volcanic aerosols and solar irradiance). The second ensemble provides various possible representations of a “natural” climate without or with only minor human influences. In these experiments, an estimate of the anthropogenic contribution to observed SST conditions is calculated and removed. Both ensembles are comprised of members that are run with slightly different (perturbed) initial conditions. In these ACE experiments, the prescribed SSTs imposed mean that the attribution statement is conditioned on the current climate state.
We also refer to HAPPI (Half a Degree Additional warming, Prognosis, and Projected Impacts) simulations (Mitchell et al., 2017). These are decade long simulations of various scenarios, including the present day (2006–20015) driven by observed SSTs and sea ice, and a historicalNat simulation and stabilized at higher warming thresholds (1.5 and 2 ∘C warmer than pre-industrial). Again, each ensemble member within an experiment suite differs from others based on initial weather conditions.
We next discuss the specifics of how these model datasets have been used in previous studies to determine attribution statements of observed Australian extremes, though note that other methodological approaches are also suitable for EEA using these models. We first detail a set of CMIP5-based attribution analyses focused on quantifying changes in the risk of Australian seasonal-scale events that were the most extreme in the observational record. Second, we discuss single-model attribution approaches. The suitability and application of these methods to other events and regions are discussed in Sect. 5.
3.1 Multi-model ensemble (CMIP5)
3.1.1 Model evaluation
Different CMIP5 models were included in ensembles used to analyse observed Australian extremes, based on data availability at the time of publication and the ability of models to capture critical aspects of observed climate. In the CMIP5-based attribution examples discussed in Sect. 4, a subset of available models was used that ideally facilitates the determination of meaningful attribution statements about causes of changes in the odds of an event. That is, models ideally capture the right mechanisms, with realistic frequency and characteristics, necessary to reproduce the event under consideration (Christidis et al., 2013a), and a sufficiently large ensemble of numerous models was available for analysis. Several approaches have been applied for assessing the validity of model simulations for Australian seasonal-scale extremes, although note that there are numerous evaluation procedures we do not discuss here.
In one example examining Australia-wide summer temperatures (Lewis and Karoly, 2013a), models were selected based on their skill in capturing interannual variability in Australian summer temperatures. First, a time series of Australia areal-average observed summer temperatures was calculated from the Australian Water Availability Project (AWAP) gridded dataset from 1910 to the present (Jones et al., 2009). Using a bootstrap resampling procedure with replacement, 2000 time series were synthesized from 10-year blocks of observed data. For each synthetic time series, a standard deviation was calculated and a spread of standard deviations determined. This resampling procedure was then applied to all available CMIP5 historical simulations to calculate simulated standard deviations of Australian summer temperatures for model years 1911–2005 (the period of modelled–observed overlap). In instances where models contributed more than one realization to a CMIP5 experiment (e.g. r1i1p1, r2i1p1), data were assessed collectively for all available realizations. Next, this study compared observed and simulated data using a Perkins skill score (Perkins et al., 2007). The Perkins score is defined as
where n is the number of bins used to calculate the PDF, Zo is the frequency of observed values, and Zm is the frequency of simulated values in a given bin. This skill score measures the common area between modelled and observed distributions (see Fig. 1). For each model, skill scores below 0.5 indicated physically unrealistic instances, where models capture less than 50 % of variations in Australian average annual mean temperature.
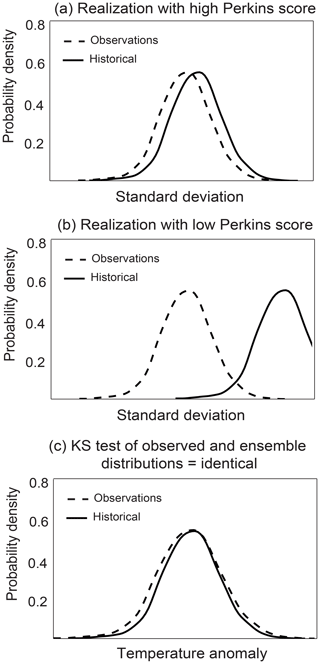
Figure 1Conceptual representation of approaches to evaluating CMIP5 models, showing a visual comparison of a high (a) and low (b) Perkins skill scores, and identical hypothetical observed and simulated distributions of temperature anomalies (c).
Further Australia EEA studies used a two-sided Kolmogorov–Smirnov test (KS) to compare modelled distributions of variables with observed ones. For example, a study of the consecutive record-breaking spring temperatures experienced in Australian in 2013/2014 used KS tests to compare temperatures in CMIP5 historical simulations from various models to observed Australian annual average temperatures. Only models where the distributions (estimated using a kernel density function) were statistically indistinguishable (p=0.05) were used for analysis.
These CMIP5 model selection steps were modified in subsequent Australia EEA studies, based on the event analysed. Analysis of the 2010–2012 eastern Australian extreme rainfall required that models capture several elements of observed climate variability (Lewis and Karoly, 2014a). Here, a larger set of evaluation criteria was applied to CMIP5 models to investigate this extreme event with a more complex climatological context than previous studies focused on large-scale long-duration temperature extremes (see Fig. 2). In this case, CMIP5 models were selected for analysis based on (1) their representation of monthly surface air temperature variability in the NINO3.4 region, (2) their representation of Australian rainfall variability and (3) rainfall amount associated with ENSO conditions (e.g. teleconnected relationship). This study considered simulated values to be physically realistic where values were in the 5th–95th percentile window of observed values determined using a bootstrap resampling method, whereby 10 000 time series of 80-year length were generated from observed anomalies. Models were only included where they are realistic compared to observation for all three criteria.
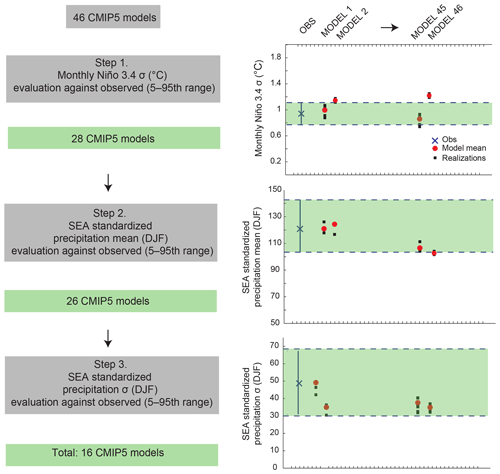
Figure 2Schematic of the three-step CMIP5 model evaluation used for examining influences on heavy rainfall of 2010–2012. Left-hand side shows flowchart evaluation steps (for the NINO3.4 index and south-eastern Australian (SEA) rainfall in December–February (DJF)) and the number of models suitable, and right-hand side demonstrates evaluation of model realizations against observations for the metrics considered.
3.1.2 FAR calculation and uncertainty assessment
Australian EEA studies using CMIP5 models have employed FAR values as a means to quantify the change in probability of an observed event that can be assigned to anthropogenic climate forcings (e.g. greenhouse gases) (see Lewis and Karoly, 2013, 2014b). The calculation of a FAR value first requires that an event be precisely defined and a threshold of exceedance determined. Various studies have defined extreme events differently from the examples highlighted here and have also demonstrated that attribution statements can be sensitive to the spatio-temporal scales used to define the event (Angélil et al., 2014; Cattiaux and Ribes, 2018; Uhe et al., 2016). Hence, each application of this EEA approach must consider event definitions and the potential sensitivity of FAR values to definitions.
Seasonal-scale Australia temperature analyses defined a threshold of the second most extreme entry in the observed record (Lewis and Karoly, 2013a, 2014b). This temperature anomaly (ΔT2) was then used rather than the most extreme anomaly (ΔT1) to avoid selection bias of using this precise value and to provide an inherently conservative analysis. Other CMIP5-based analyses have used a suite of thresholds to determine FAR values. When examining the record heavy 2010–2012 precipitation across eastern Australia, Lewis and Karoly (2014a) calculated FAR values based on exceeding a series of thresholds defined by the observed precipitation mean (“average”), 1 standard deviation above normal (“heavy”) and 2 standard deviations above normal (“extreme”).
FAR values compare the probability of an event exceeding the pre-defined threshold (e.g. ΔT2) in each processed CMIP5 experiment. In these studies, the CMIP5-derived FAR was calculated using Eq. (1), with either the historical simulations (most recent years 1976–2005) or rcp8.5 simulations (years 2006–2020) as the forced (“ALL”) state, and either the historicalNat or piControl simulations as the reference (“NAT”) state. In the Australia studies highlighted in Sect. 4, FAR values were reported for various CMIP5 experiment comparisons. The 2013 record annual and spring temperature study by Lewis and Karoly (2014b) provided FAR values determined by comparing CMIP5 historical years 1976–2005 with historicalNat/piControl combined data, in addition to FAR values determined by comparing these same naturally forced reference simulations with rcp8.5 years 2006–2020. This comparison demonstrated the change in FAR values (i.e. risk of extremes attributable to anthropogenic forcings) through time.
The probabilities in Eq. (1) are determined through several possible approaches. The count-based estimate simply determines the number of times the defined threshold was exceeded, relative to the total sample size. Alternatively, probabilities can be calculated as the area under the fitted distribution exceeding the event threshold, compared to the entire distribution (Lewis and Karoly, 2013b). Distributions can be fitted using a kernel density function or a fitted generalized extreme value (GEV) model which is suited to examining rare events.
Studies also attempt to assess uncertainty in FAR calculations. For each experiment, only a single FAR value is obtained for each realization of each model. A bootstrap resampling procedure was applied in this set of CMIP5-based studies to evaluate the uncertainty associated with FAR estimated. In determining the FAR values associated with the 2012/2013 Australian summer temperatures, each distribution of temperature was bootstrap resampled 10 000 times (using in each iteration sub-samples of all years from only 50 % of available model simulations) and a distribution of FAR values was then calculated (Lewis and Karoly, 2013b). This calculated distribution of 10 000 FAR values represents the uncertainty associated with using different models and provides a basis for communicating FAR ranges. This 2012/2013 study, for example, reported both the median and 10th percentile FAR values, meaning they are exceeded by 90 % of the values in the bootstrapped FAR distributions. These are described respectively as “best estimate” and “very likely” values. By providing both the best estimate (median) and very likely (10th percentile) values, an estimate of the uncertainty in FAR values is conveyed.
3.2 Single-model ensemble (CESM)
Perkins et al. (2014) used the 21-member version of CESM to determine the anthropogenic signal behind heatwave intensity and frequency during the 2012/2013 Australian summer. The total number of heatwave days (heatwave frequency), as well as the hottest heatwave day (heatwave intensity) (see Perkins and Alexander, 2013), were computed using the excess heat factor heatwave metric (Nairn and Fawcett, 2013). This metric was used since it underpins operational heatwave forecasts in Australia. Areally averaged heatwave frequency and intensity for the 2012/2013 austral summer were extracted from AWAP (Jones et al., 2009). The frequency of each metric was compared via the forced and controlled simulations, as outlined above. Over the period 1984–2012, heatwave intensity increased by 2-fold, and frequency by 3-fold, relative to the counterfactual climate.
The calculations of FAR using a multi-member single-model ensemble are similar to those described for CMIP5. That is, evaluations based on the similarity between the observed and model distributions, such as the Kolmogorov–Smirnov test, are employed, however for each individual realization against the observations. Since Australian climate extremes occurring after 2005 have been analysed by this approach, the historical and rcp8.5 realizations were spliced together. Previous attribution studies using CESM have found no significant difference between each CESM realization and the observational dataset that includes the extreme event of interest (Perkins-Kirkpatrick et al., 2019a; Perkins et al., 2014; Perkins and Gibson, 2015). A more detailed evaluation of the weather systems that drive Australian heatwaves is provided by Perkins and Gibson (2015), with the model performing adequately across all 21 members.
After evaluation, bootstrapping with replacement is performed 10 000 times to create new samples of the counterfactual and factual simulations, each of which are 50 % the size of the original. This allows for uncertainties in model sampling. For each bootstrapped iteration, the observed extreme of interest is isolated and its frequency compared in the control and forced samples, resulting in a distribution of 10 000 FAR values. As a conservative measure, the 10th percentile FAR value is reported. This allows for 90 % confidence that the true FAR value based on CESM is at least as big as this value.
It is notable that small atmospheric perturbations initiate substantial differences in the resolving climate variability (see Fig. 3), and, therefore, within the extremes themselves. For example, Fischer et al. (2013) demonstrated that the spatially aggregated distribution of changes in numerous extremes in CESM is comparable to that in CMIP5. Ranges in regional heatwave trends also significantly vary across CESM realizations (Perkins and Fischer, 2013). The representation of synoptic systems, which ultimately drive extreme events over short timescales, also demonstrates striking differences among CESM realizations (Schaller et al., 2018). Thus, using just one or a few realizations from a given model may not be an accurate estimate of how an extreme event is represented or changing within that model. Internal variability, and the physical mechanisms it induces, can dampen or enhance the actual climate signal of that model for the extreme event of interest, particularly when FAR is estimated for decadal-based time slices. Employing a large number of realizations for a single model therefore comprehensively samples the influence of internal variability and provides a robust FAR estimate for that individual model.
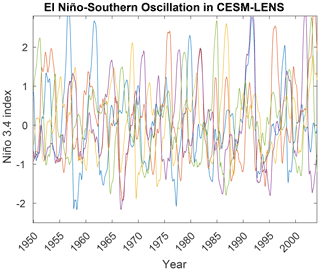
Figure 3Example of the different realizations of ENSO, as defined by the NINO3.4 index, in a version of CESM. For comparison, a subset of only 5 of 35 available realizations is shown using different colours which demonstrate vastly different ENSO conditions in realizations differing based on perturbed initial atmospheric conditions.
We next detail examples that have employed the methods described above to examine observed weather and climate extremes in Australia.
4.1 Multi-model ensemble (CMIP5) and combined approaches
4.1.1 2012/2013 Australian summer temperatures
The first study employing CMIP5 detection and attribution experiments to quantitatively assess the relative influence of different climatic forcings on an observed extreme was focused on the record hot Australia-wide 2012/2013 summer (Lewis and Karoly, 2013a). The Australia-wide mean temperatures from December 2012 to February 2013 were the hottest in the observed high-quality record (extending to 1910), with average conditions exceeding the observed 1911–1940 mean by 1.32 ∘C. This study compared the probability of extremes such as 2012/2013 in historical and rcp8.5 experiments with historicalNat and piControl experiments, using the threshold of the second highest mean (Tmean), maximum (Tmax) and minimum (Tmin) summer temperatures observed.
By using FAR values to compare the likelihood of extreme Australia-wide summer temperatures between the CMIP5 experiments, this analysis showed that it was very likely (>90 % confidence) there was at least a 2.5 times increase in the odds of extreme heat (Tmean) due to human influences using simulations to 2005 (with a best estimate FAR value of 0.72) and a 5-fold increase in this risk using simulations for 2006–2020 (with a best estimate FAR value of 0.87). The observed event is expected to occur 1-in-16 years without anthropogenic influences, in 1-in-6 years in the historical experiment and 1-in-2 years in the rcp8.5 experiment.
4.1.2 2016 Great Barrier Reef bleaching
Quantitative attribution using CMIP5 models also contributed to a later study analysing factors affecting Great Barrier Reef (GBR) bleaching (Lewis and Mallela, 2018). In 2016, the GBR endured a significant bleaching event: 93 % of the northern, 700 km stretch of coral was bleached, and by June, >60 % of this coral had been killed in association with heat stress. As coral bleaching is intimately connected to heat stress, amongst other risk factors, this 2018 attribution study attempted to determine the change in likelihood of anomalous heat in the Coral Sea region that houses the GBR. As coral heat stress is accumulated through both the magnitude and duration of temperatures above a threshold, several sea surface temperature metrics were investigated, including immediate heat stress, antecedent heat stress and degree heating weeks (DHWs).
For each metric, probabilities of exceedance were compared in CMIP5 detection and attribution experiments. Results varied depending on the metric. There was a significant increase in the likelihood of extreme January–March temperature anomalies when anthropogenic forcings are included (median FAR =0.85; 10th percentile FAR =0.80). More notably, the 2016 observed conditions for combined antecedent and coincident SST anomalies (DJF, together with March–May conditions) do not occur in CMIP5 simulations without anthropogenic forcings (FAR =1). While this study examined a suite of climatic, environmental and biotic factors, the CMIP5-based attribution permitted the statement to be made that “Anthropogenic greenhouse gases likely increased the risk of the extreme Great Barrier Reef bleaching event through anomalously high sea surface temperature and the accumulation of thermal stress”.
4.1.3 2010–2012 Australian heavy rainfall
The extreme heavy precipitation of 2010–2012 occurring over Australia was also examined from an attribution perspective (Lewis and Karoly, 2014a). Over this period, eastern Australia experienced its heaviest ever 2-year accumulated rainfall. Rainfall records were broken on daily through to seasonal timescales for large spatial regions. The heavy rainfall and disastrous accompanying flooding were coincident with two strong, consecutive La Niña events, which are typically associated with enhanced rainfall in the eastern Australian rainfall. Using five CMIP5 climate models, King et al. (2013) examined the anthropogenic influence on rainfall totals and maximum consecutive 5 d rainfall (Rx5day) across south-eastern Australia in 2012. This analysis determined that there was little robust change in the risk of extreme rainfall events between the 1861–1890 and 1976–2005 periods. Overall, the magnitude of cool sea surface temperatures in the NINO3.4 region was found to have a greater effect on Rx5day compared to the magnitude of anomalously warm local SSTs.
A further study by Lewis and Karoly (2014a) examined this period of record heavy precipitation using CMIP5 models in combination with two sets of simulations conducted as part of the ACE initiative, in which ensembles of simulations are produced representing the recent climate with, and then without, the effects of human influences. This study explored the heavy rainfall in several defined Australia regions on multiple seasonal to multi-year timescales, and results were broadly in agreement with King et al. (2013). The approach used aimed to determine the robustness of FAR values for the 2010–2012 heavy Australia rainfall to changes in the attribution framework. Results showed that attribution statements for the rainfall events were variable, with FAR values sensitive to the attribution parameters considered, including event thresholds, regions and seasons. Furthermore, estimates of the attributable change in rainfall risk depended on the model datasets considered. This study argued that consideration of model outputs from several datasets (e.g. CMIP5 and ACE) was useful for establishing robust attribution statements for extreme rainfall events.
4.2 Single-model ensemble (CESM) and combined approaches
4.2.1 2014 Australian May heatwave
Analysis of the 2014 Australian May heatwave demonstrated a 23-fold increase in experiencing an event of similar concurrent length and magnitude over the period 1995–2020 (Perkins and Gibson, 2015). This study was focused on a 19 d heatwave during 8–26 May 2014, areally averaged for Australia, that had a magnitude of +2.52 ∘C above 1961–1990 May temperatures. The event was compared between the control and forced CESM simulations (see Fischer et al., 2013) using the methods described above. The 23-fold increase (FAR =0.96) over 1995–2020 is almost double the 12-fold increase (FAR =0.92) detected for the earlier period 1975–1994.
4.2.2 2017/2018 Tasman Sea marine heatwave
In analysing the 2017/2018 Tasman Sea marine heatwave, the 35-member ensemble of CESM was used in conjunction with CMIP5 to compare the two approaches (Perkins-Kirkpatrick et al., 2019a). This was to address whether different amounts of anthropogenic warming were detected via the two ensembles. During November 2017–January 2018, sea surface temperatures (SSTs) were 1.7 ∘C higher over the greater Tasman Sea compared to 1961–1990. The occurrence rates in percentages were computed for the current (2008–2027) and future (2041–2060) periods. This approach was used since the methods described above would have resulted in FAR assessments of 1, as this particular event never occurred in the counterfactual world. Interestingly, it was found that the event magnitude was so rare that it did not occur until approximately 2035 in CESM. Within the future climates, a signal of a 56 % occurrence rate was higher in CESM than the 41 % occurrence rate in CMIP5. Moreover, the human influence behind the initiating atmospheric pressure system was also analysed. During November 2017, mean sea level pressure just off south-eastern Australia was 5.2 hPa stronger than the 1961–1990 monthly average. A small anthropogenic signal was found in the CESM ensemble (an increase of 3 % in future climates), though not CMIP5. Thus, combining the results across the two approaches, it was concluded that while climate change rendered the 2017/2018 Tasman Sea marine heatwave virtually impossible in the absence of anthropogenic climate change, natural climate variability was an important influence on the physical mechanism which initiated the event.
4.3 Expansion to future extremes under varying levels of global mean warming
When the Paris Agreement was drafted in December 2015, with the aim of limiting global warming to well below 2 ∘C above pre-industrial levels and with a preferential aim of 1.5 ∘C global warming (UNFCCC, 2016), there was little understanding of the characteristics of the climate at 1.5 ∘C global warming. The extension of the existing event attribution framework to future 1.5 and 2 ∘C global warming levels helped to expand our knowledge of these climates and how contemporary extremes would change in frequency in these climates. The subsequent studies were well utilized in policy documents, including the United Nations Intergovernmental Panel on Climate Change (IPCC) Special Report on 1.5 ∘C published in October 2018.
Here we briefly discuss the “time-slicing” techniques used to analyse extremes in future warmer worlds using a multi-model ensemble such as CMIP5. The CMIP5 ensemble, and in particular the simulations run under different representative concentration pathways, are designed to investigate transient climate change projections under plausible greenhouse gas emissions scenarios. Careful application of a “time-slicing method” (James et al., 2017) allows for analysis of 1.5 and 2 ∘C climates in transient states, which are quite likely to be the outcome in the real world given emissions trajectories and existing emissions reduction pledges.
In the CMIP5 models, a pre-industrial climate baseline may be defined from a choice of experiments including the control simulations or historical simulations with anthropogenic influences removed. Subsequently, time slices from individual climate model projections can be extracted (see Fig. 4 for a schematic). For example, King et al. (2017) extracted all model years within decades where the global-average temperature was 1.3–1.7 ∘C above the equivalent model natural baseline and defined this as their 1.5 ∘C world. Similarly, the 2 ∘C world was defined as years within decades where the global average temperature was 1.8–2.2 ∘C above the equivalent model natural baseline. When extracting the years within the 1.5 and 2 ∘C worlds, there are choices that can be made around
-
the use of all RCPs or just a subset of scenarios,
-
the definition of a model pre-industrial baseline,
-
and the temperature range and length of the averaging window.
For (1), if the difference that is being inferred between the 1.5 and 2 ∘C worlds is strongly influenced by the rate of global warming (e.g. sea level rise), then the results will differ between scenarios and they should not be combined. In the case of Australian temperature and precipitation means and extremes there was little evidence of a difference due to the rate of global warming through 1.5 and 2 ∘C (King et al., 2017). For (2) it has been shown that the use of different approximations for pre-industrial baselines (e.g. late 19th century observations/historical simulations or historicalNat simulations for the 20th century) can make a difference of as much as 0.1–0.2 ∘C between baselines (Hawkins et al., 2017) and, therefore, in the estimate of anthropogenic warming to date (Haustein et al., 2017). This choice will influence the timing within the projections when the global-average temperature will have warmed by 1.5 or 2 ∘C. For (3), the use of shorter averaging windows and/or narrower temperature ranges to define the warmer worlds will decrease sample sizes. Given the aim is to best simulate the world at 1.5 ∘C anthropogenic warming, some leeway must be given here to incorporate natural interannual and interdecadal variability that can result in departures in global-average surface temperature of as much as 0.2 ∘C in individual years due to phenomena such as ENSO and the Interdecadal Pacific Oscillation.
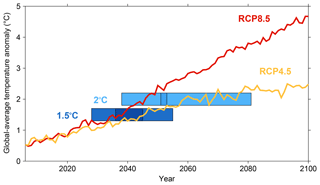
Figure 4Schematic illustrating the time-slice method. Global-average annual temperature anomalies (relative to 1901–2005 historicalNat average) are shown for ACCESS1.3 rcp8.5 and rcp4.5 for 2006–2100. The boxes show the years which are extracted from each RCP to construct the 1.5 ∘C global warming world (dark blue) and the 2 ∘C world (light blue). The overlap window between the RCPs is indicated with a darker colour.
This framework for analysis was then applied in King et al. (2017) to examine Australian extremes under the Paris Agreement global warming limits. They extended existing attribution analyses for events like the record hot “Angry summer” of 2012/2013 (Lewis and Karoly, 2013) to examine their likelihoods under future warming scenarios. Consistent with Lewis and Karoly (2013), King et al. (2017) found a large increase in the likelihood of hot Australian summers exceeding the previous record, from ∼3 % chance per year in a pre-industrial climate to ∼44 % chance per year in the current world. In future, the probability of having summer temperatures that are considered extreme contemporarily rises further, to the point that they become commonplace and cooler than average. In a 1.5 ∘C world, the likelihood of hot Australian summer temperatures is ∼57 % and in a 2 ∘C world it is ∼77 %. This result supports the statement that there is a substantial benefit to limiting global warming for Australia in terms of reduced frequency of heat extremes. The analysis was extended to other contemporarily extreme events, such as the hot sea temperatures in the Coral Sea associated with Great Barrier Reef coral bleaching in 2016 and the heavy rainfall in Queensland in December 2010.
This framework illustrates the utility of the CMIP5 ensemble and event attribution methodology beyond examining extreme events only in the current climate context. Analysis of the 1.5 and 2 ∘C warmer worlds results in an important contribution to our understanding of these possible future climates and their implications. We also note that other model setups have been used to examine specifically the changing nature of climate extremes in warmer worlds, including atmosphere-only multi-model ensembles (HAPPI) (Mitchell et al., 2017) and single coupled model ensembles (BRACE) (Sanderson et al., 2017). Whilst CMIP5 transient future simulations have general use for examining possible future climates, these are arguably of less utility in analysing the precise implications of specific global warming targets (hence the development of frameworks such as HAPPI). These warming target-based model datasets have recently also been applied to Australian extremes (e.g. Lewis et al., 2017a).
5.1 Multi-model ensemble (CMIP5)
The CMIP5-based event attribution methodology described above is useful for estimating the roles of anthropogenic and natural climate influences in extreme events. By making comparisons between model simulations representing the current climate and that of a counterfactual world without anthropogenic influences, the effect humans have had on extreme events may be quantified. Overall, the presented method is limited in its applications for several key reasons.
First, the CMIP5-based approaches are best suited to large spatio-temporal events (sub-continental to continental spatial-scale extreme events on daily to multi-year timescales), rather than short-duration or small-scale events that likely require large ensemble sizes and/or finer horizontal resolution. Second, the CMIP5 method is useful in sampling the anthropogenic attributable signal across varying climate model configurations. This means that differences in model physics, parameterization schemes, the simulation/exclusion of certain physical mechanisms that drive extreme events, as well as climate model sensitivity, are accounted for when quantifying FAR values. However, most models employed using this method are represented by just one or at most a few realizations of the same experiment (e.g. historical, historicalNat, rcp8.5). Therefore, the influence of internal variability is unlikely fully accounted for, especially at the individual model level.
5.2 Single-model ensemble (CESM)
Using a large ensemble of a single model in attribution assessments should not currently be seen as a substitute to other approaches, particularly when this type of ensemble is limited to, at best, a handful of individual models. Rather, this approach is complementary to existing methods, by including a robust anthropogenic estimate from plausible realizations of fully coupled internal climate variability. For this to be effective, evaluation of the model must be performed so its simulation of observed conditions is verified. Indeed, should enough individual models provide multi-member ensembles similar to CESM, future versions of this approach, when performed over an ensemble of these models, could replace the current CMIP5-style approach. Since relatively few realizations of each model are provided in CMIP5, the effect of internal variability of each model on resulting FAR assessments is not necessarily adequately accounted for. This needs to be taken into consideration as the FAR methodology evolves in the future, and we note that an increasing number of large ensembles are becoming available for analysis (see Kirchmeier-Young et al., 2019; Maher et al., 2019; Parker et al., 2017).
6.1 Recommendations for future application of GCM-based approaches
This paper has outlined a cogent examination of recent extreme temperature and precipitation events in Australia from an extreme event attribution perspective using GCMs (and some ESMs). The models used in these examples are not conditioned to specific atmospheric or oceanic states and hence data are available and accessible for use. The methodologies can be applied to developing attribution statements for extreme events occurring elsewhere, provided that event-specific model evaluation is performed first. For precipitation-related extremes in particular, we recommend that rigorous model evaluation is necessary prior to attribution and that multiple model-based approaches are combined (for example, the combination of GCM- and AMIP-based results). In some extreme event cases, conditional EEA model frameworks that are conditioned by specific boundaries (SSTs or seasonal forecasts) may be more robust (National Academies of Sciences, Engineering, and Medicine, 2016).
There are some important overall limitations of the methods we have presented here. Firstly, while these studies provide robust evaluations of models used against observed climates, they typically employ singular analytical approaches. That is, one set of models (CMIP5 or CESM) was used to examine the characteristics of observed extremes for most of the reviewed studies, which may affect the attribution statement provided. Independent re-examination of other extreme event attribution studies found that attribution statements are broadly similar for extreme temperature events upon re-evaluation, but disagree for many extreme precipitation events (Angélil et al., 2017). Similarly, the Lewis and Karoly (2014a) study discussed here found that aspects of attributing the heavy Australian rainfall over 2010–2012 to specific causes were model dependent. While the set of studies we have reviewed provide robust evidence of anthropogenic influences on observed Australia temperature-related extremes, in combination with further results (e.g. Knutson et al., 2014; Christidis et al., 2013a), a comprehensive analysis of uncertainty has not yet been undertaken. We suggest a combination of approaches should be used in future attribution studies.
We also note that the approaches are present-day focused and consider only anthropogenic influences on climate events already observed. As the world is projected to further warm under growing greenhouse emissions, it is also helpful to extend the extreme event attribution framework to understand extremes in future, warmer climates. This was future-focused analysis demonstrated in the time-slicing approach. For EEA studies quantifying the human influence in temperature extremes using an attribution framework, there is a general trend towards a greater degree of confidence in the results of temperature-related studies. For some temperature extremes, studies have identified events that are virtually impossible without anthropogenic influences (FAR values of one) (Imada et al., 2018; Knutson et al., 2018; Lewis and Karoly, 2014b; Walsh et al., 2018; Perkins-Kirkpatrick et al., 2019). More recent studies are increasingly presenting risk ratio (RR) rather than FAR values, as a clearer communication of attribution statements (see discussion in National Academies of Sciences, Engineering, and Medicine, 2016) and in light of the rapid saturation of FAR values for some event types (Harrington et al., 2018). Reflecting on the results of the studies presented here and these FAR issues, we suggest that further applications of the methods we have outlined present risk ratio () rather than FAR values.
6.2 Future directions
While these studies have provided comprehensive evidence of the factors (anthropogenic and natural) contributing to specific observed extremes, several unexplored research directions should be noted. As the validity of all event attribution statements depends on the representation of observed climates and variability in models, a systematic analysis of uncertainty in attribution statements in the Australian context would be useful for providing recommendations around robust attribution statements. While a larger sample of independent models could make up for some of the under-representation of internal climate variability, emerging evidence suggests that the variability in some extremes in a multi-member ensemble for a single climate model encompasses a considerable portion of the CMIP5 ensemble (Fischer et al., 2013; Perkins-Kirkpatrick and Gibson, 2017). Thus, if multi-member ensembles were available for all coupled climate models, a truer estimate on the influence of internal variability, in addition to the structural uncertainties motioned above, would be accounted for in resulting attribution assessments. While currently, multi-member ensembles of each climate model in a repository like CMIP5 are not available, largely due to computational and storage constraints, such a project is being undertaken as part of US CLIVAR (Climate Variability and Predictability Program (https://www.earthsystemgrid.org/dataset/ucar.cgd.ccsm4.CLIVAR_LE.html, last access: 1 July 2019). We also note that the approaches presented here estimate FAR uncertainty using bootstrap resampling approaches. This technique, however, is noted to perform poorly in quantifying statistical uncertainty, and comprehensive studies performed elsewhere argue for the implementation of more sophisticated statistical methods (Paciorek et al., 2018).
The temperature and precipitation-based studies highlighted here effectively demonstrate how anthropogenic climate change is already affecting extremes and is likely to do so further under the Paris Agreement warming levels. However, these studies only broach this key research area, focusing for the most part on large spatial- and temporal-scale extremes such as seasonal heat. Further studies exploring the contributing factors to complex climatological events (such as the emerging 2018 drought in New South Wales), compound events (such as recent heatwaves co-occurring with catastrophic fire weather in eastern Australia) or event impacts (such as on the Great Barrier Reef ecosystem or on human health) would be scientifically valuable as well as useful to a range of stakeholders and policy-makers. Future work attributing Australia's weather and climate extremes will target these complex events and impacts using CMIP6, in addition to other GCM-based datasets.
We have presented no new analyses here. AWAP, CMIP5 (https://cmip.llnl.gov/cmip5/data_getting_started.html, last access: 4 December 2018), C20C (https://portal.nersc.gov/c20c/data.html, last access: 4 December 2018), CESM (http://www.cesm.ucar.edu/models/ccsm4.0/model_esg/, last access: 4 December 2018) and HAPPI (http://www.happimip.org/happi_data/, last access: 4 December 2018) data are all publicly available datasets and available for download.
All the authors participated in the writing and editing of the manuscript. SCL developed Figs. 1 and 2, SEP Fig. 3 and ADK Fig. 4.
The authors declare that they have no conflict of interest.
This article is part of the special issue “Methods for detection and attribution of anthropogenic climate change”. It is not associated with a conference.
We acknowledge the support of the NCI facility in Australia and we acknowledge the World Climate Research Programme's Working Group on Coupled Modelling, which is responsible for CMIP, and we thank the climate modelling groups for producing and making available their model output. We also acknowledge the CESM Large Ensemble Community Project and supercomputing resources provided by NSF/CISL/Yellowstone. We thank the Bureau of Meteorology, the Bureau of Rural Sciences and CSIRO for providing the Australian Water Availability Project (AWAP) data.
Sophie C. Lewis is funded through the Australian Research Council (DE160100092). Sarah E. Perkins-Kirkpatrick is funded through the Australian Research Council (FT170100106). Andrew D. King is funded through the Australian Research Council (DE180100638).
This paper was edited by Francis Zwiers and reviewed by two anonymous referees.
Angélil, O., Stone, D. A., Tadross, M., Tummon, F., Wehner, M., and Knutti, R.: Attribution of extreme weather to anthropogenic greenhouse gas emissions: Sensitivity to spatial and temporal scales, Geophys. Res. Lett., 41, 2150–2155, https://doi.org/10.1002/2014GL059234, 2014.
Angélil, O., Stone, D., Wehner, M., Paciorek, C. J., Krishnan, H., and Collins, W.: An independent assessment of anthropogenic attribution statements for recent extreme temperature and rainfall events, J. Climate, 30, 5–16, https://doi.org/10.1175/JCLI-D-16-0077.1, 2017.
Bureau of Meteorology: Australia's wettest two-year period on record, 2010–2011, Spec. Clim. Statement 38, available at: http://www.bom.gov.au/climate/current/statements/scs38.pdf (last access: 16 July 2019) 10 February 2012.
Bureau of Meteorology: Australia's warmest September on record, Spec. Clim. Statement 46, available at: http://www.bom.gov.au/climate/current/statements/scs46.pdf (last access: 16 July 2019), October 2013a.
Bureau of Meteorology: Extreme heat in January 2013, Special Climate Statement 43, 1–19, available at: http://www.bom.gov.au/climate/current/statements/scs43e.pdf (last access: 16 July 2019), February 2013b.
Bureau of Meteorology: Extreme Rainfall and Flooding in Coastal Queensland and New South Wales, Spec. Clim. Statement 44, available at: http://www.bom.gov.au/climate/current/statements/scs44.pdf (last access: 16 July 2019), 18 February 2013c.
Bureau of Meteorology: Annual Climate Report 2013, available at: http://www.bom.gov.au/climate/annual_sum/2015/Annual-Climate-Report-2015-HR.pdf (last access: 16 July 2019), 2014.
Bureau of Meteorology: Australia' s warmest autumn on record, Special Climate Statement 52, available at: http://www.bom.gov.au/climate/current/statements/scs56.pdf (last access: 16 July 2019), 25 June 2016a.
Bureau of Meteorology: State of the Climate 2016, available at: http://www.bom.gov.au/state-of-the-climate/2016/ (last access: 16 July 2019), 2016b.
Cattiaux, J. and Ribes, A.: Defining single extreme weather events in a climate perspective, B. Am. Meteorol. Soc., 99, 1557–1568, https://doi.org/10.1175/BAMS-D-17-0281.1, 2018.
Christidis, N., Stott, P. A., Karoly, D. J., and Ciavarella, A.: An Attribution Study of the Heavy Rainfall Over Eastern Australia in March 2012, B. Am. Meteorol. Soc., 94, S58–61, 2013.
Fischer, E. M., Beyerle, U., and Knutti, R.: Robust spatially aggregated projections of climate extremes, Nat. Clim. Change, 3, 1033–1038, https://doi.org/10.1038/nclimate2051, 2013.
Harrington, L. J., Otto, F. E. L., and Res, E.: Adapting attribution science to the climate extremes of tomorrow, Environ. Res. Lett., 13, 123006, https://doi.org/10.1088/1748-9326/aaf4cc, 2018.
Haustein, K., Allen, M. R., Forster, P. M., Otto, F. E. L., Mitchell, D. M., Matthews, H. D., and Frame, D. J.: A real-time Global Warming Index, Sci. Rep., 7, 1–6, https://doi.org/10.1038/s41598-017-14828-5, 2017.
Hawkins, E., Ortega, P., Suckling, E., Schurer, A., Hegerl, G., Jones, P., Joshi, M., Osborn, T. J., Masson-Delmotte, V., Mignot, J., Thorne, P., and Van Oldenborgh, G. J.: Estimating changes in global temperature since the preindustrial period, B. Am. Meteorol. Soc., 98, 1841–1856, https://doi.org/10.1175/BAMS-D-16-0007.1, 2017.
Herring, S. C., Hoerling, M. P., Peterson, T. C., and Stott, P. A.: Explaining extreme events of 2013 from a climate perspective, B. Am. Meteorol. Soc., 95, S1–S96, 2014.
Herring, S. C., Hoerling, M. P., Kossin, J. P., Peterson, T. C., and Stott, P. A.: Explaining extreme events of 2014 from a climate perspective, B. Am. Meteorol. Soc., 96, S1–S172, https://doi.org/10.1175/BAMS-ExplainingExtremeEvents2014.1, 2015.
Herring, S. C., Hoell, A., Hoerling, M. P., Kossin, J. P., III, Shreck, C. J., and Stott, P. A.: Explaining extreme events of 2015 from a climate perspective, B. Am. Meteorol. Soc., 97, S1–S145, https://doi.org/10.1175/BAMS-D-12-00021.1, 2016.
Herring, S. C., Christidis, N., Hoell, A., Kossin, J. P., Schreck Iii, C. J., Stott, P. A., Quan, X. W., Hoerling, M., Smith, L., Perlwitz, J., Zhang, T., Hoell, A., Wolter, K., and Eischeid, J.: Explaining extreme events of 2016 from a climate perspective, B. Am. Meteorol. Soc., 99, 54–59, https://doi.org/10.1175/BAMS-D-17-0118.1, 2018.
Imada, Y., Shiogama, H., Takahashi, C., Watanabe, M., Mori, M., Kamae, Y., and Maeda, S.: Climate Change Increased the Likelihood of the 2016 Heat Extremes in Asia, B. Am. Meteorol. Soc., 99, S97–S101, https://doi.org/10.1175/BAMS-D-17-0109.1, 2018.
James, R., Washington, R., Schleussner, C. F., Rogelj, J., and Conway, D.: Characterizing half-a-degree difference: a review of methods for identifying regional climate responses to global warming targets, Wires Clim. Change, 8, https://doi.org/10.1002/wcc.457, 2017.
Jones, D. A., Wang, W., and Fawcett, R.: High-quality spatial climate data-sets for Australia, Aust. Meteorol. Ocean., 58, 233–248, 2009.
Kay, J. E., Deser, C., Phillips, A., Mai, A., Hannay, C., Strand, G., Arblaster, J. M., Bates, S. C., Danabasoglu, G., Edwards, J., Holland, M., Kushner, P., Lamarque, J.-F., Lawrence, D., Lindsay, K., Middleton, A., Munoz, E., Neale, R., Oleson, K., Polvani, L., and Vertenstein, M.: The Community Earth System Model (CESM) Large Ensemble Project: A Community Resource for Studying Climate Change in the Presence of Internal Climate Variability, B. Am. Meteorol. Soc., 96, 1333–1349, https://doi.org/10.1175/BAMS-D-13-00255.1, 2015.
King, A. D., Lewis, S. C., Perkins, S. E., Alexander, L. V., Donat, M. G., Karoly, D. J., and Black, M. T.: Limited evidence of anthropogenic influence on the 2011–12 extreme rainfall over Southeast Australia, B. Am. Meteorol. Soc., 94, 55–58, 2013.
King, A. D., Klingaman, N. P., Alexander, L. V., Donat, M. G., Jourdain, N. C., and Maher, P.: Extreme rainfall variability in Australia: Patterns, drivers, and predictability, J. Climate, 27, 6035–6050, https://doi.org/10.1175/JCLI-D-13-00715.1, 2014.
King, A. D., Karoly, D. J., and Henley, B. J.: Australian climate extremes at 1.5 ∘C and 2 ∘C of global warming, Nat. Clim. Change, 7, 412–416, https://doi.org/10.1038/nclimate3296, 2017.
Kirchmeier-Young, M. C., Zwiers, F. W., and Gillett, N. P.: Attribution of extreme events in Arctic Sea ice extent, J. Climate, 30, 553–571, https://doi.org/10.1175/JCLI-D-16-0412.1, 2017.
Kirchmeier-Young, M. C., Gillett, N. P., Zwiers, F. W., Cannon, A. J., and Anslow, F. S.: Attribution of the Influence of Human-Induced Climate Change on an Extreme Fire Season, Earth's Future, 7, 2–10, https://doi.org/10.1029/2018EF001050, 2019.
Knutson, T., Zeng, F., and Wittenberg, A.: Seasonal and annual mean precipitation extremes during during 2013: A U.S. focused analysis, B. Am. Meteorol. Soc., 95, S19–S23, 2014.
Knutson, T. R., Kam, J., Zeng, F., and Wittenberg, A. T.: CMIP5 Model-based Assessment of Anthropogenic Influence on Record Global Warmth During 2016, B. Am. Meteorol. Soc., 99, S11–S15, https://doi.org/10.1175/BAMS-D-17-0104.1, 2018.
Lewis, S. C. and Karoly, D. J.: Anthropogenic contributions to Australia's record summer temperatures of 2013, Geophys. Res. Lett., 40, 3708–3709, https://doi.org/10.1002/grl.50673, 2013.
Lewis, S. C. and Karoly, D. J.: Are estimates of anthropogenic and natural influences on Australia's extreme 2010–2012 rainfall model-dependent?, Clim. Dynam., 45, 679–695, https://doi.org/10.1007/s00382-014-2283-5, 2014a.
Lewis, S. C. and Karoly, D. J.: The Role of Anthropogenic Forcing in the Record 2013 Australia-Wide Annual and Spring Temperatures, B. Am. Meteor.ol Soc., 95, S31–S34, 2014b.
Lewis, S. C. and Mallela, J.: A Multifactor Analysis of the Record 2016 Great Barrier Reef Bleaching, B. Am. Meteorol. Soc., S144–S149, https://doi.org/10.1175/BAMS-D-17-0074.1, 2018.
Lewis, S. C., King, A. D., and Mitchell, D. M.: Australia's Unprecedented Future Temperature Extremes Under Paris Limits to Warming, Geophys. Res. Lett., 44, 9947–9956, https://doi.org/10.1002/2017GL074612, 2017a.
Lewis, S. C., Karoly, D. J., King, A. D., Perkins, S. E., and Donat, M. G.: Mechanisms Explaining Recent Changes in Australian Climate Extremes, in: Climate Extremes, American Geophysical Union (AGU), 249–263, 2017b.
Maher, N., Milinski, S., Suarez-Gutierrez, L., Botzet, M., Kornblueh, L., Takano, Y., Kröger, J., Ghosh, R., Hedemann, C., Li, C., Li, H., Manzini, E., Notz, D., Putrasahan, D., Boysen, L., Claussen, M., Ilyina, T., Olonscheck, D., Raddatz, T., Stevens, B., and Marotzke, J.: The Max Planck Institute Grand Ensemble – enabling the exploration of climate system variability, J. Adv. Model. Earth Sy., 11, https://doi.org/10.1029/2019MS001639, online first, 2019.
Maher, P. and Sherwood, S. C.: Disentangling the multiple sources of large-scale variability in Australian wintertime precipitation, J. Climate, 27, 6377–6392, https://doi.org/10.1175/JCLI-D-13-00659.1, 2014.
Min, S. K., Cai, W., and Whetton, P.: Influence of climate variability on seasonal extremes over Australia, J. Geophys. Res.-Atmos., 118, 643–654, https://doi.org/10.1002/jgrd.50164, 2013.
Mitchell, D., AchutaRao, K., Allen, M., Bethke, I., Beyerle, U., Ciavarella, A., Forster, P. M., Fuglestvedt, J., Gillett, N., Haustein, K., Ingram, W., Iversen, T., Kharin, V., Klingaman, N., Massey, N., Fischer, E., Schleussner, C.-F., Scinocca, J., Seland, Ø., Shiogama, H., Shuckburgh, E., Sparrow, S., Stone, D., Uhe, P., Wallom, D., Wehner, M., and Zaaboul, R.: Half a degree additional warming, prognosis and projected impacts (HAPPI): background and experimental design, Geosci. Model Dev., 10, 571–583, https://doi.org/10.5194/gmd-10-571-2017, 2017.
Moss, R. H., Edmonds, J. A., Hibbard, K. A., Manning, M. R., Rose, S. K., van Vuuren, D. P., Carter, T. R., Emori, S., Kainuma, M., Kram, T., Meehl, G. A., Mitchell, J. F. B., Nakicenovic, N., Riahi, K., Smith, S. J., Stouffer, R. J., Thomson, A. M., Weyant, J. P., and Wilbanks, T. J.: The next generation of scenarios for climate change research and assessment, Nature, 463, 747–756, https://doi.org/10.1038/nature08823, 2010.
Nairn, J. and Fawcett, R.: Defining heatwaves: heatwave defined as a heat-impact event servicing all communiy and business sectors in Australia, CAWCR Tech. Rep. No 060, 84 pp., available at: https://www.cawcr.gov.au/technical-reports/CTR_060.pdf (last access: 17 July 2019), 2013.
National Academies of Sciences, Engineering, and Medicine: Attribution of Extreme Weather Events in the Context of Climate Change, Washington, DC, The National Academies Press, https://doi.org/10.17226/21852, 2016.
Paciorek, C. J., Stone, A., and Wehner, M. F.: Quantifying statistical uncertainty in the attribution of human influence on severe weather, Weather and Climate Extremes, 20, 69–80, https://doi.org/10.1016/j.wace.2018.01.002, 2018.
Parker, H. R., Lott, F. C., Cornforth, R. J., Mitchell, D. M., Sparrow, S., and Wallom, D.: A comparison of model ensembles for attributing 2012 West African rainfall, Environ. Res. Lett., 12, https://doi.org/10.1088/1748-9326/aa5386, 2017.
Perkins, S., Lewis, S., King, A., and Alexander, L.: Increased simulated risk of the hot Australian summer of 2012/13 due to anthropogenic activity as measured by heatwave intensity and frequency, B. Am. Meteorol. Soc., 95, S34–37, https://doi.org/10.1175/1520-0477-95.9.s1.1, 2014.
Perkins, S. E. and Alexander, L. V.: On the measurement of heat waves, J. Climate, 26, 4500–4517, https://doi.org/10.1175/JCLI-D-12-00383.1, 2013.
Perkins, S. E. and Fischer, E. M.: The usefulness of different realizations for the model evaluation of regional trends in heat waves, Geophys. Res. Lett., 40, 5793–5797, https://doi.org/10.1002/2013GL057833, 2013.
Perkins, S. E. and Gibson, P. B.: Increased risk of the 2014 Australian May heatwave due to anthropogenic activity, B. Am. Meteorol. Soc., 96, https://doi.org/10.1175/BAMS-D-15-00074.1, 2015.
Perkins, S. E., Pitman, A. J., Holbrook, N. J., McAneney, J., Change, C., and Frontiers, R.: Evaluation of the AR4 climate models' simulated daily maximum temperature, minimum temperature, and precipitation over Australia using probability density functions, J. Climate, 20, 4356–4376, https://doi.org/10.1175/JCLI4253.1, 2007.
Perkins, S. E., Argüeso, D. and White, C. J.: Relationships between climate variability, soil moisture, and Australian heatwaves, J. Geophys. Res.-Atmos., 120, 8144–8164, https://doi.org/10.1002/2015JD023592, 2015.
Perkins-Kirkpatrick, S. E. and Gibson, P. B.: Changes in regional heatwave characteristics as a function of increasing global temperature, Sci. Rep., 7, 1–12, https://doi.org/10.1038/s41598-017-12520-2, 2017.
Perkins-Kirkpatrick, S. E., King, A. D., Cougnon, E. A., Grose, M. R., Oliver, E. C. J., Holbrook, N. J., Lewis, S. C., and Pourasghar, F.: The role of natural variability and anthropogenic climate change in the 2017/18 Tasman Sea marine heatwave, B. Am. Meteorol. Soc., 100, S105–S110, https://doi.org/10.1175/BAMS-D-18-0116.1, 2019.
Queensland Floods Commission of Inquiry: Queensland Floods Commission of Inquiry Final Report, 658, available at: http://www.floodcommission.qld.gov.au/publications/final-report/ (last access: 3 April 2019), 2012.
Sanderson, B. M., Xu, Y., Tebaldi, C., Wehner, M., O'Neill, B., Jahn, A., Pendergrass, A. G., Lehner, F., Strand, W. G., Lin, L., Knutti, R., and Lamarque, J. F.: Community climate simulations to assess avoided impacts in 1.5 and 2 ∘C futures, Earth Syst. Dynam., 8, 827–847, https://doi.org/10.5194/esd-8-827-2017, 2017.
Schaller, N., Sillmann, J., Anstey, J., Fischer, E. M., Grams, C. M., and Russo, S.: Influence of blocking on Northern European and Western Russian heatwaves in large climate model ensembles, Environ. Res. Lett., 13, 054015, https://doi.org/10.1088/1748-9326/aaba55, 2018.
Stone, D. A. and Allen, M. R.: The end-to-end attribution problem: From emissions to impacts, Climatic Change, 71, 303–318, https://doi.org/10.1007/s10584-005-6778-2, 2005.
Stott, P. A., Christidis, N., Otto, F. E. L. L., Sun, Y., Vanderlinden, J. P., Oldenborgh, G. J. Van, Vautard, R., Storch, H. Von, Walton, P., Yiou, P., Zwiers, F. W., van Oldenborgh, G. J., Vautard, R., von Storch, H., Walton, P., Yiou, P., and Zwiers, F. W.: Attribution of extreme weather and climate-related events, Wires Clim. Change, 7, 23–41, https://doi.org/10.1002/wcc.380, 2016.
Taylor, K. E., Stouffer, R. J., and Meehl, G. A.: An overview of CMIP5 and the experiment design, B. Am. Meteorol. Soc., 93, 485–498, https://doi.org/10.1175/BAMS-D-11-00094.1, 2012.
Uhe, P., Otto, F. E. L., Haustein, K., van Oldenborgh, G. J., King, A. D., Wallom, D. C. H., Allen, M. R., and Cullen, H.: Comparison of methods: Attributing the 2014 record European temperatures to human influences, Geophys. Res. Lett., 43, 8685–8693, https://doi.org/10.1002/2016GL069568, 2016.
UNFCCC: Paris Agreement, Article 2, Vol. 01415, 2016.
Van Dijk, A. I. J. M., Beck, H. E., Crosbie, R. S., De Jeu, R. A. M., Liu, Y. Y., Podger, G. M., Timbal, B., and Viney, N. R.: The Millennium Drought in southeast Australia (2001–2009): Natural and human causes and implications for water resources, ecosystems, economy, and society, Water Resour. Res., 49, 1040–1057, https://doi.org/10.1002/wrcr.20123, 2013.
Walsh, J. E., Thoman, R. L., Bhatt, U. S., Bieniek, P. A., Brettschneider, B., Brubaker, M., Danielson, S., Lader, R., Fetterer, F., Holderied, K., Iken, K., Mahoney, A., McCammon, M., and Partain, J.: The High Latitude Marine Heat Wave of 2016 and Its Impaacts on Alaska, B. Am. Meteorol. Soc., 99, S39–S43, https://doi.org/10.1175/BAMS-D-17-0105.1, 2018.
- Abstract
- Introduction
- Model descriptions
- Specifics of EEA approaches
- Examples of application to Australian extremes
- Ideal applications and specific limitations
- Conclusions
- Code and data availability
- Author contributions
- Competing interests
- Special issue statement
- Acknowledgements
- Financial support
- Review statement
- References
- Abstract
- Introduction
- Model descriptions
- Specifics of EEA approaches
- Examples of application to Australian extremes
- Ideal applications and specific limitations
- Conclusions
- Code and data availability
- Author contributions
- Competing interests
- Special issue statement
- Acknowledgements
- Financial support
- Review statement
- References