the Creative Commons Attribution 4.0 License.
the Creative Commons Attribution 4.0 License.
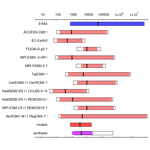
Formally combining different lines of evidence in extreme-event attribution
Friederike E. L. Otto
Clair Barnes
Sjoukje Philip
Sarah Kew
Geert Jan van Oldenborgh
Robert Vautard
Event attribution methods are increasingly routinely used to assess the role of climate change in individual weather events. In order to draw robust conclusions about whether changes observed in the real world can be attributed to anthropogenic climate change, it is necessary to analyse trends in observations alongside those in climate models, where the factors driving changes in weather patterns are known. Here we present a quantitative statistical synthesis method, developed over 8 years of conducting rapid probabilistic event attribution studies, to combine quantitative attribution results from multi-model ensembles and other, qualitative, lines of evidence in a single framework to draw quantitative conclusions about the overarching role of human-induced climate change in individual weather events.
- Article
(1117 KB) - Full-text XML
- BibTeX
- EndNote
Extreme-event attribution aims at answering questions of whether and to what extent anthropogenic climate change – or other drivers external to the climate system – have altered the likelihood and intensity of extreme weather events. Over recent years, methods to quantify this change based on historic weather observations and climate model data have been developed (National Academies of Sciences, Engineering, and Medicine, 2016; Stott et al., 2016; van Oldenborgh et al., 2021) alongside more qualitative approaches or storylines, conditional on aspects of the event, e.g. the thermodynamics (Shepherd, 2016; Vautard et al., 2016; Jézéquel et al., 2018). Exact quantification of the role of climate change strongly depends on the geographical and temporal definitions of the event (Leach et al., 2020) but also on the observational datasets and the climate model simulations included in the study. In order to provide assessments that incorporate these sources of uncertainty, methodologies have been developed that use a range of models and modelling frameworks as well as multiple simulations from the same model that differ by initial conditions, thus allowing for systematic uncertainties from differences between models to be taken into account (model uncertainty) as well as uncertainties arising from internal variability (sampling uncertainties) (Philip et al., 2020; Li and Otto, 2022). If the aim of an attribution study is not only to highlight uncertainties but also to provide an overarching attribution statement, the estimated changes in intensity and likelihood from different data sources need to be combined. While it might be appropriate in some cases to provide a simple average, this will in many cases not represent the statistical properties and underlying scientific understanding correctly, because uncertainties will differ for each line of evidence. Below in Sect. 2 we discuss how these can be taken into account to provide a formal synthesis of changes in likelihood and intensity by combining different observational and model datasets, taking sampling and systematic uncertainties into account.
Such formal syntheses do not however allow for information not contained in the data. In many cases we do have more knowledge than the data represent, which usually only provides one line of reasoning and which can be complemented by knowledge about physical processes, emerging trends in the future or studies based on other methods and models that corroborate a particular interpretation of the statistical data. Section 3 provides a detailed discussion of how a systematic assessment of these additional lines of evidence leads to different interpretations of the statistical results and thus the overarching attribution statement. We close with a discussion of limitations and future research needs (Sect. 4).
A standard method of probabilistic attribution using time series of observations is to fit the data to a statistical distribution – typically a generalised extreme-value distribution – that varies with a covariate that describes global warming, such as the smoothed global mean surface temperature (GMST) (Philip et al., 2020; Otto, 2023; Chen et al., 2021). Because all of the observed warming in the global mean temperature is attributable to anthropogenic climate change (Eyring et al., 2021), this can be translated into an estimate of the human influence on this extreme if other local factors such as land use changes do not play a role. As the latter can never be known perfectly in the real world, true attribution requires one to repeat this analysis for climate models, in which such factors play no part and so changes in probability or intensity can be directly linked to anthropogenic emissions. As in other uses of climate model information, it is important to use a large number of climate models with a range of framings wherever possible: multi-model ensembles give information about model uncertainties from the model spread, while multi-member ensembles can provide information about uncertainty arising from the choice of initial conditions (Stocker et al., 2013; National Academies of Sciences, Engineering, and Medicine, 2016; Hauser et al., 2017). Each individual model is evaluated on the extreme under study (van Oldenborgh et al., 2021). If the model deviates too much from the observations in the statistical properties of these extremes or selected meteorological properties, it is not considered further.
For each observational series and each model that passes the evaluation, we compute the factor of change in the probability that an event of similar or greater magnitude will occur (known as the probability ratio, PR) and the change in intensity of the event (ΔI) for a fixed change in the GMST covariate: this reflects the change in likelihood and intensity due to anthropogenic climate change. Along with the best estimate of these two measures, PR and ΔI, a bootstrapping procedure is used to estimate the upper and lower bounds of a 95 % confidence interval for each (Philip et al., 2020). A synthesis of each measure is done separately.
The bootstrapped PRs are first transformed to be more normally distributed by taking a logarithm, and their distribution is assumed to be Gaussian. Depending on the statistical model fitted, the change in intensity ΔI can be a shift, in which case we assume that the bootstrapped values are normally distributed, or a percentage change, in which case a logarithmic transformation is applied to make them more normally distributed. When dependence on the smoothed GMST is linear, the absolute change in intensity per unit increase in the covariates remains constant for events of any magnitude. Percentage changes are best used when the dependence on the smoothed GMST is exponential, e.g. for heavy precipitation, in which case the relative change in intensity per unit change in the covariates is constant.
The synthesis is performed in three steps. First, the observational estimates are combined in a single best estimate with confidence intervals including uncertainties from natural variability and (if there are multiple observed datasets) representativity errors. Next, we do the same for the model uncertainties, accounting for the effect of model natural variability and model spread. Finally, these two sub-results are combined to get the overall attribution result.
We now describe the assumptions about the structure of the observations and models and the relationships between them that underlie the synthesis algorithm. To simplify the explanation, the following development is presented in terms of the means and standard deviations of the normally distributed (log-transformed) PRs or intensity changes. In this simplified symmetric model, we assume that the change estimated from the ith observational dataset has mean μi and variance . Similarly, the change obtained from the jth climate model is assumed to be normally distributed with mean μj and variance . This is illustrated in Fig. 1, which shows examples from different studies. A description of the datasets used in each panel can be found in the references mentioned in the caption. Intervals associated with these distributions appear as coloured bars (light blue for observations, light red for models) against individual datasets in the examples in Fig. 1. The same procedure is applied to each of the normalised measures, so we do not distinguish between them in the description below. In practice, it is easier to work directly with the bootstrapped confidence intervals, and the uncertainty ranges that come out of the extreme-value fits are often very asymmetric around the central value. Working with these confidence bounds and accommodating asymmetry in the intervals is discussed in Sect. 2.4. As noted above, we recommend that the changes to be synthesised be estimated over a common increase in GMST (or other covariates of interest). However, it is possible to synthesise responses to changes of different magnitudes or over different time periods; a discussion of the necessary adjustments can be found in Appendix A.
2.1 Observations
The light-blue bars in Fig. 1 represent 95 % confidence intervals estimated by bootstrapping, corresponding to approximately μi±1.96σi for the ith model. Assuming that the observational data product is a reliable representation of the true climate and that the statistical model is a good fit to the data, these intervals represent natural variability and sampling uncertainty within each dataset. However, the observations cannot be assumed to be perfectly representative of the “real” extreme under study, and additional uncertainty may arise from a poor fit in the statistical model. We therefore introduce an additional term to account for this “representation error”. Because the observational datasets are all based on the same realisation of reality over similar or at least overlapping time intervals, they can be assumed to have strongly correlated natural variability; we therefore assume that differences between the best estimates {μi} are due to representation errors, which are in theory independent of the natural variability. Natural variability in this case encompasses both unforced variability as well as variability forced by drivers not assessed in the study, e.g. large-scale modes of variability like the AMO or PDO (Atlantic Meridional Oscillation or Pacific Decadal Oscillation). The representation error may be large because the observational data products disagree on the strength of the climate change signal or because the chosen statistical model is unable to provide a reliable estimate of the quantity of interest. The latter issue is particularly likely to arise when estimating return periods and probability ratios for very extreme or unprecedented events, which may arise from physical processes that are different from those driving less rare events and so may appear to be drawn from an entirely different statistical distribution; typically, estimates of the change in intensity are more robustly estimated and the representation error is smaller. In reality, the natural variability sampling will include observational errors and vice versa. As the natural variability estimated by bootstrapping is usually very large, we take the assumption to be justified in practice and note that it can only produce an overly conservative statement, not overconfidence in the results. We further assume that there is no systematic bias in the representation errors, so that they are normally distributed with mean zero and variance and that this representation error, which is estimated using the spread of the best estimates {μi}, is roughly the same for all the observational datasets. If one or more of the datasets were known a priori to have a much larger representation error than the others, it would be better to exclude them from the analysis. If only one observational dataset is available for the analysis, there is no way of estimating , and this source of additional uncertainty is disregarded. We acknowledge that the assumption that there is no systematic bias in the representation error may not be fully justified, particularly when estimating probability ratios using a generalised extreme value (GEV) distribution: model parameters are usually fitted using maximum likelihood estimation, and this is known to induce a bias in estimated return periods and therefore probability ratios, particularly when using short observational records (Zeder et al., 2023). In the absence of a reliable method of estimating the magnitude of such a bias, we keep the simplifying assumption but note that, in this situation, the bootstrapped uncertainties around the probability ratios are likely to be much higher than those around the corresponding changes in intensity. This should be highlighted when interpreting the results, as discussed in Sect. 3.
In this framework, the overall change estimated using the nobs observational datasets is assumed to follow a normal distribution with mean μobs and variance accounting for both natural variability and representation errors, with
where
where ni is the number of observational datasets included in the analysis.
2.2 Models
For the model estimates we again assume that the total variability can be decomposed into contributions from natural variability and representation error, so that . Our aim is to combine the model results in a single estimate by taking a weighted average, where the weight wj represents our degree of confidence in model j; this is an approach used in meta-analyses to combine the results from multiple studies in a single minimal-variance result. Defining appropriate weights is notoriously difficult, and in many climate model applications an agnostic approach is used, giving equal weight to each model. Other weighting schemes have mainly been tested with respect to their performance of large-scale future warming (e.g. Merrifield et al., 2020) and thus might not necessarily be most appropriate in the context of attribution. Abramowitz et al. (2019) suggest that similar models should be down-weighted, but there is no consensus on how this should be carried out, especially when models show very different behaviour; experience has shown that most ensembles in use for attribution consist of a few different models, and their similarities can usually be neglected. Similarly, weighting models according to their performance is not a trivial task (Sansom et al., 2013; Haughton et al., 2015). It is not uncommon for a few models to estimate outlying values with high uncertainty, in which case an unweighted mean would likely result in an overall estimate with extremely high variance and risks producing statistically insignificant results, underestimating the role of climate change (Lloyd and Oreskes, 2018). While in science avoiding type-1 errors is usually treated as the highest priority, in the case of climate change attribution this could lead to dangerously false feelings of security; thus, we use the minimal-variance scheme to reduce the risk of type-2 errors. As a first step, we note that the obviously unrealistic models have already been dropped in the model evaluation stage prior to synthesis (Philip et al., 2020), which is equivalent to giving those models a weight of 0. We also note that, while inverse-variance weighting is used to combine the model outputs, the final combination of the observational and model results may use either a weighted or unweighted mean, as discussed in Sect. 3.
For the models that pass evaluation, we use a precision-weighted average in which the weights wj are determined by the inverse square of the total variance for each model, so that
However, the situation differs from that described in Sect. 2.1 above in that the chaotic nature of the weather means that atmospheric fields usually decorrelate within about 2 weeks: if the models are initialised more than 2 weeks before the event, their realisations of natural variability are expected to be uncorrelated1, and we cannot simply average the estimates of {σj} to estimate the contribution from natural variability, as we did for the observations in Eq. (2). Because the natural variability is not shared between models, it is no longer straightforward to estimate the relative contribution from natural variability – and hence the shared representation error – in the way that we could for the observational datasets, and the model representation error is therefore not known. However, we now have two estimates of the variability: the variances estimated from the climate models and the spread of the best estimates {μj}. A logical step is then to compare these two estimates: if the two are roughly equal, then the spread of {μj} is compatible with {σj}: the natural variability explains the spread between the models, and other sources of variability are assumed to be much smaller. If the differences between {μj} are too large to be explained by natural variability alone, the representation error is significant. If an ensemble of runs is available, the ensemble spread may be used to estimate the natural variability for an individual model. However, in many cases only one model realisation is available, so in general the method suggested here will be required. We therefore impose the constraint that the sum of the ratios between these two estimates of variability (denoted as χ2) should be equal to the total degrees of freedom (that is, the number of models nj−1) and estimate by solving
Initially, is assumed to be zero: if , then the spread of the best estimates μj is smaller than would be expected from natural variability alone, and we assume that the differences between the model estimates are due to internal variability only. However, if , then is found by numerical optimisation and added to for each model: these are the white uncertainty boxes around the individual model bars in Fig. 1b. As with the observations, we assume that there is no common bias in the model representation error; while this may not be true for models within a single ensemble or single framing, the use of models from different framings – for example, SST-forced, regional and coupled global models, as suggested by Philip et al. (2020) – is expected to reduce the effect of biases due to shared modelling assumptions between related families of models.
Having estimated and hence {wj}, we can now calculate
This is illustrated by the bright-red bar in Fig. 1a, b. Note that this uncertainty may not represent the full model uncertainty, which can be larger than the spread due to biases that are shared by all the models.
2.3 Combination
We finally combine the observational and model results.
We apply two different weighting approaches to combining models with observations. An unweighted average gives observations and models equal weight, as described below, and a variance-based weighting that is commonly applied in statistics but rarely when combining climate models. Given that we combine models and observations and that observations tend to have a very large variance simply due to the very small sampling, an unweighted combination could have overly large variance and in many cases end up producing statistically insignificant results (see the magenta and white bars in Fig. 1a) and thus lead to overly conservative estimates of the role of climate change (Lloyd and Oreskes, 2018). While in science avoiding type-1 errors is usually treated as the highest priority, in the case of climate change attribution this could lead to dangerously false feelings of security. Thus, to avoid type-2 errors, we chose a weighted variance scheme. This can however become overconfident (see the magenta and white bars in Fig. 1b) when some models have a small sampling uncertainty or systematically miss certain processes. We thus also calculate an unweighted mean. A level of expert judgement is required to interpret between the two, as discussed in Sect. 3.
If models and observations are clearly incompatible – that is, if – the conclusion has to be that the representation error in the models is so large that the two numbers cannot be compared. A common cause of this is that an essential mechanism with a trend is missing in the models, such as the effects of irrigation on heat waves in India (van Oldenborgh et al., 2018) or the likely effect of roughness changes on wind trends (Vautard et al., 2019), as seen in Fig. 1c.
If the difference and uncertainty are compatible, the overall synthesis is constructed as the weighted average of these two estimates from observations and models, with weights again given by the inverse total variances
where
However, the weighted average neglects the unknown difference between the model spread and the true model uncertainty. This is especially acute when, for example, there is one model with much more data and therefore much smaller sampling variability than the others: if the results for that model fall within the range of results from other models with larger uncertainties, the algorithm will conclude that the model spread is compatible with natural variability and will therefore return a very narrow confidence interval in the synthesis, which is likely to be unduly influenced by that particular model. It is obvious that the true model error is larger, but there is no information available to estimate it from the multi-model ensemble results. In principle, the model evaluation conducted prior to the attribution could provide an estimate, but this is not a trivial task and requires detailed knowledge about the individual models, which is not necessarily easily available.
The only comparison we have left to give an estimate of the true model uncertainty is with the observations. We therefore also give the unweighted average of the means and variances from the observations and models as an alternative synthesis:
The unweighted average disregards the differing precision of the two estimates and emphasises their systematic uncertainties. Only judgement of the fidelity of the models in the extreme under study can determine which of the two averages describes the final attribution statement better. In Sect. 3 we discuss ways to decide whether to use the weighted or unweighted synthesis.
2.4 Asymmetric confidence intervals
In practice, when carrying out the synthesis, we obtain bootstrapped estimates of the upper and lower bounds of 95 % confidence intervals, which may be very asymmetric around the central value (as in Fig. 2, for example). A crude way to propagate this information is to consider the probability distribution function (PDF) in each case to be the weighted sum of two half-normal distributions that meet at the modal value but have different variances; the representation errors are still assumed to be symmetric. In the analysis of the observations this simply means that the upper and lower bounds of the confidence intervals, denoted as and respectively, are calculated separately, so that
σrep is found using Eq. (2) and added in quadrature to these estimates to obtain a 95 % confidence interval that incorporates both natural variability and representation errors into the observational datasets:
The interval from to is plotted as a dark-blue bar on either side of μobs in the synthesis plots shown in Fig. 1. The white bars added to each observational dataset account for representation uncertainty in the same way, showing
When we estimate the weights used to combine the models in Eq. (3), the standard deviations are derived from the widths of the corresponding 95 % confidence intervals, with
and similarly Eq. (4) becomes
where 𝟙 is an indicator function taking the value 1 if the condition in the subscript is true and 0 otherwise.
Finally, to combine the estimates from the models and observations, the weights are redefined as
Then,
These quantities are indicated by the magenta bars in Fig. 1. Finally, we find the unweighted average of the observational and model-averaged confidence bounds measured as deviations from the direct average of the best estimates of the observational and model averages, and combined in quadrature (white box around or overlapping the magenta weighted average in Fig. 1) they are
2.5 Interpreting synthesis plots
In Fig. 1, three examples are shown. Figure 1a shows the probability ratio for 3 d extreme precipitation in the Seine basin in May–June (Philip et al., 2018). The meteorology of large-scale mid-latitude precipitation was captured well by the models used, which resulted in good model evaluation (not shown) and is reflected in the synthesis in the low model spread that is well within the observational uncertainty in the absence of white bars. We therefore quoted the weighted average and stated that the probability of an event like this increased by at least 40 % (lower bound of the overall synthesis, given by the magenta bar) but probably more than doubled, as the best estimate is very consistent between observations and models. In contrast, Fig. 1b shows the change in potential evaporation in western Ethiopia (Kew et al., 2021), where both the different “observations” (re-analyses) and models diverge widely. Given our knowledge of model performance in this part of the world, we think that the model uncertainty is even larger than the spread indicated by the white boxes, so we consider the unweighted white box synthesis statement to be more reliable than the weighted colour bar. We use this for at least the upper bound. For the lower bound, physical constraints provide additional knowledge. For example, the fact that increasing temperatures strongly increase evaporation should be taken into consideration, as discussed in Sect. 3. Figure 1c shows an example where observed and modelled trends are incompatible. The models do not include changes in roughness and possibly other relevant factors that cause the strong decline in storminess over land in the observations (Vautard et al., 2019). Thus, no synthesis is shown.
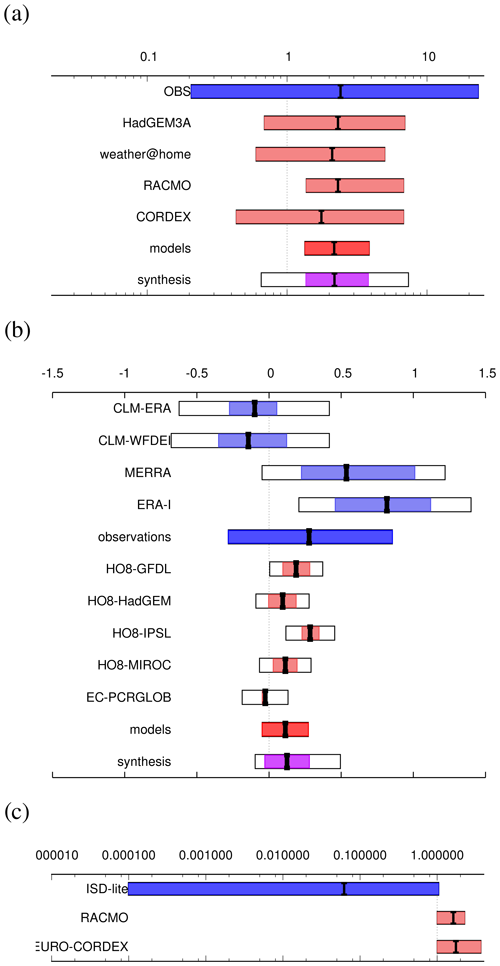
Figure 1Example synthesis plots. Panel (a) is dominated by natural variability (PR of 3 d extreme precipitation in the Seine basin, Philip et al., 2018), panel (b) is dominated by model spread (change in potential evaporation in western Ethiopia, Kew et al., 2021), and panel (c) shows incompatible values (PRs of extreme wind speeds similar to Cyclone Friederike, Vautard et al., 2019).
Finally, Fig. 2a shows an example where observations and models both show the same result qualitatively but disagree quantitatively by orders of magnitude. The example shown here is a heat wave in Madagascar (https://www.worldweatherattribution.org/extreme-poverty-rendering-madagascar-highly-vulnerable-to-underreported-extreme-heat-that-would-not-have-occurred-without-human-induced-climate-change/, last access: 29 October 2024), but similar results are commonly found in many heat-wave studies (van Oldenborgh et al., 2022). As this is a known deficiency in models when underestimating the observed trend in heat extremes, we only report the lower bound.
2.6 Impossible extreme events
Especially in recent years extreme events have occurred, most notably heat waves that lie beyond the upper limit of what the non-stationary model deems possible in a pre-industrial climate, leading to infinite PRs: typically this affects the best estimate of the PR or the bootstrapped upper bound of the confidence interval but can occur even in the bootstrapped lower bound. In these cases, in order to still numerically combine results in a synthesis and visualise the results, it is necessary to translate infinity into a numerical value. In earlier studies, where only a few upper bounds were infinite, missing PRs were arbitrarily set to 10 000. However, this practice, when applied to more than the odd upper bound, distorts the relative weights wobs and wmod given to the observations and models in the synthesised result, because both depend on the range (Eq. 11), as do the weights {wj} assigned to the individual climate models (Eq. 9). The synthesis results are therefore sensitive to the choice of replacement value. We first discuss the theoretical effects of this replacement and subsequently give recommendations for how to handle infinite values in practice. However, it is important to highlight that this is only in order to enable the visualisation of the results. The exact or approximate numbers are meaningless for probability ratios approaching infinity, and any conclusion would need to clearly state that the event in question is too rare to quantify in a counter-factual climate.
2.6.1 theoretical effects of replacing infinite values
We now discuss the theoretical effects of replacing infinite values in the upper bounds with finite ones, which provides a framework for deciding how infinite values should be replaced. While this gives an indication of the expected effect of replacing different values, the sensitivity of the results will depend on where the infinite values occur, how many infinite values occur and the finite value into which they are translated. In general, the aim should be to select a replacement value large enough that the resulting weights from Eqs. (9) and (11) reflect the level of relative confidence that we would wish to place in each element.
Suppose that the nv of the upper bounds estimated from the observations is infinite and truncated to some value . Then, from Eqs. (1)–(2), μobs and will be unaffected while, from Eq. (6), will be inflated by approximately . The synthesised variance will therefore be inflated by and the unweighted variance by .
The situation is slightly different for models, which are weighted according to their precision rather than equally, as the observations are. From Eq. (9), the relative weight wj assigned to the jth model is determined by ; thus, if only some of the models have infinite upper bounds replaced by , then those models are down-weighted and effectively ignored by the synthesis algorithm. If all of the models have infinite upper bounds replaced by some very large v, then the models are given roughly equal weights in the model synthesis (dark-red bar), but from Eq. (11) the total weight wmod will be very low compared to wobs, and so the models will contribute very little to the synthesis (magenta bar), although the upper bound of the unweighted synthesis will be increased to reflect the additional uncertainty.
If v is not much larger than the other finite bounds, then the models are still down-weighted, but not to the extent that they are effectively dropped from the synthesis altogether. The choice of v is therefore critical in determining how the models and observations are weighted to produce the overall synthesis statement. The replacement value v may therefore be chosen to reflect reduced confidence in the model projections by down-weighting their contribution to the synthesis. Recall that we work with log-transformed probability ratios, which are assumed to be approximately normally distributed; we might therefore choose to set the replacement value , reflecting an approximate 6σ interval above the best estimate based on the width of the finite lower interval. By doing this, we halve the weight wj that would be assigned to model j if the confidence interval were symmetric, because
This approach can easily be modified to reflect differing levels of confidence in the information from those climate models that produce infinite upper bounds.
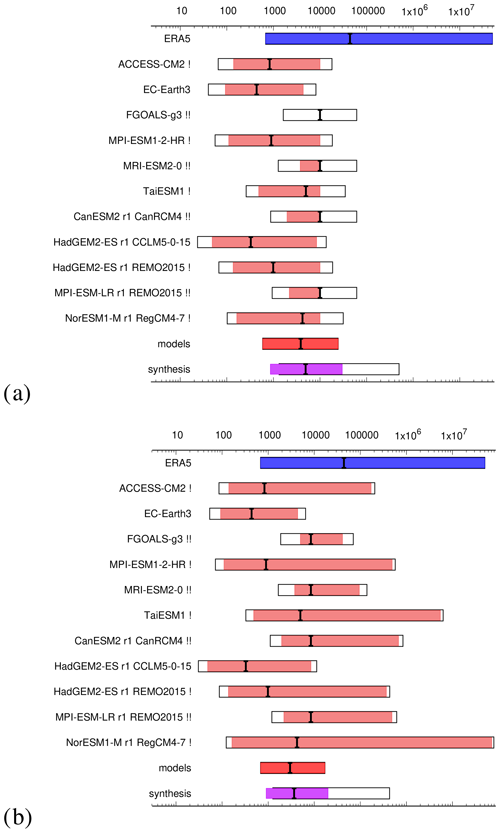
Figure 2PR of the 2023 heat wave in Madagascar where observations and models are incompatible in order of magnitude with numerical replacements for (a) infinite values in the synthesis routine of 10 000 and (b) the 6σ replacement discussed in Sect. 2.6.2. Models with one infinite value replaced are flagged with “!”; models with two or more infinite values replaced are flagged with “!!”.
2.6.2 Replacing infinite values in practice
Given the theoretical considerations above, the main objective when replacing infinite values in any given study is to keep the relative weights of the individual datasets consistent after replacement. The actual numbers, as mentioned above, are comparably meaningless anyway. We thus suggest in cases where only the upper bound is infinite to calculate the numerical upper bound for the visualisation as 6 times the standard deviation, as proposed above. This value is chosen as it is a common indicator of virtually impossible risks in engineering contexts. When the lower bound and best estimate are finite, this can simply be calculated by adding the difference between the lower bound and the best estimate multiplied by 3 to the best estimate given that the lower bound represents a deviation of 2σ. When the best estimate is also infinite, we suggest using the highest finite upper bound from other models as the best estimate in these models and repeating the calculation above to obtain the numerical upper bounds. If the lower bound is also infinite, we choose the highest best estimate of the other models as the lower bound and proceed as above. Any models for which infinite values have been replaced with finite values should be clearly marked. In Fig. 2 we show the results of the synthesis when applying this method compared with the previously applied method of setting infinity to 10 000 for the upper bounds (and 9999 for the best estimates and 9998 for the lower bounds). The overall synthesised results are not very different with respect to the orders of magnitude, with a PR of 4920 (860–30 000) when replacing infinities with 10 000 and 3660 (906–199 000) when using the method suggested here, which keeps the relative weights according to the actual modelling results. However, the range of uncertainty captured by the second method is slightly narrower for the weighted uncertainties (magenta bar) and, crucially, given the consistency of the relative weights, a preferred methodology that represents the actual results better. For the unweighted synthesis, the upper bounds are at 497 000 and 431 000 respectively and thus identical for all practical purposes. However, all the methods are comparably arbitrary, and we can only conclude with high confidence that there is a strong trend, but we have low confidence in the exact number.
2.7 Conclusions about the formal synthesis
The formalism described here gives a practical and operational way of combining the information of various observational and model-based estimates of the PRs and changes in intensity (ΔI) of extreme events, taking into account several sources of uncertainties: natural variability in both observations and models, observation representativity and model uncertainty. The true representativity uncertainty and model uncertainty can be either smaller or larger than the spread. It can be smaller if there are values that are known to be less accurate than the others included in the synthesis, e.g. less reliable observations or a model with known deficiencies. It can be larger because the observations and models suffer from common (shared) flaws that are not reflected in the spread. However, without additional information these deviations cannot be estimated. The additional information would have to come from the observational dataset and model evaluations, but it is unclear at the moment how to best use these evaluations to weigh the results beyond the include–exclude step we have adopted. This additional knowledge can however be used after the analysis is carried out to decide whether to present the best estimate, the lower or upper bound respectively or the range as the overarching result. As this is done after the synthesis, where models are either included or excluded but not otherwise reweighted, this requires an additional step but has the advantage of making assumptions much more transparent than they would be if included in an algorithm.
The formal statistical synthesis methodology introduced here allows us to transparently combine the evidence estimated using the available climate data. The errors from insufficient sampling of variability and structural uncertainties are higher when fewer data are available. Structural uncertainty is furthermore strongly influenced by the quality of the data with respect to observations, reanalysis and the climate models used. A lot of knowledge is available beyond what can be captured with formal, quantitative or qualitative model evaluation. The synthesis thus only represents the available data, not the available knowledge. For a meaningful overarching attribution statement, both need to be combined. To do this, we qualitatively address the lines of evidence and available knowledge beyond the statistical analysis. Based on these, we create an overarching message.
3.1 Structured assessment of lines of evidence
This approach essentially follows an assessment of the following points:
-
Goodness of fit of the statistical model
-
Quality of observations, including differences between different observational datasets and uncertainties in observed trends
-
Model results, including known deficiencies of the models, the agreement between models and the agreement between observations and model averages
-
Agreement between observations and models
-
Analysis of future trends
-
Physical understanding, e.g. the Clausius–Clapeyron relationship
-
Other published research or research syntheses such as included in IPCC reports and government assessments.
The assessment of any of these points helps to judge how much confidence to put in the overarching synthesised result and whether to report lower or upper bounds, the full range or the best estimate.
For example, in the Ethiopian drought case shown in Fig. 1b, no actual observations are available, but only reanalysis data, which differ widely. Hence, the quality of the observations is low (2) and the quality of the fit (1) is also questionable, rendering the best estimate essentially meaningless. This also means that the model evaluation is conducted against low-quality data, and thus the tests are not very meaningful. The only conclusions that can be drawn in a case like this, other than “we don't know”, need to be strongly informed by physics (see below). In this example, the agreement between the best estimate of the models and observations is also poor, which means that the weighted average provides too small an uncertainty range that is not supported by what we know about the data, so in this case the white bars are judged to represent a better uncertainty estimate. In Fig. 1a, models and observations agree very well, and thus the magenta box indicating the weighted average would be the chosen result. In contrast, the observations for the example of Cyclone Friederike are actual high-quality observations showing a clear trend that is in opposition to the models, indicating that the observed trend is not driven by human-induced climate change through processes present in models.
In a recent study performed in western Asia (https://www.worldweatherattribution.org/human-induced-climate-change-compounded-by-socio-economic-water-stressors-increased-severity-of-drought-in-syria-iraq-and-iran/, last access: 28 October 2024), the model evaluation step showed that, while models represented temperatures overall well in the flat basin of the Euphrates and Tigris, this was not the case over Iran. This is not surprising, given the known difficulty of models in representing mountainous terrain well (3). This led to the overall conclusion to regard the two results with different levels of confidence: “It is important to highlight that the models represent temperatures over the river basin well, but less so over Iran with much more varied topography, hence confidence is high for the assessment over the Tigris-Euphrates basin, but medium over Iran.”
The analysis of future trends (5) is important in every study, as a qualitatively different trend from the past and present is always an indicator that any trend found up to today is not, or at least not only, driven by human-induced climate change. If however there is a significant trend in future simulations, even if it is not significant in the past, the reported result will be that a trend is emerging rather than favouring the assumption of no trend at all. An example is the 2022 floods in Pakistan (Otto et al., 2023), where an assessment of future trends led to the conclusion that “intense rainfall has become heavier as the world has warmed, [and] that climate change indeed increased the rainfall intensity up to 50 % as the best estimate in some models.”
This latter conclusion in the case of the Pakistan floods has not only been informed by an assessment of future trends, but has also been informed by the knowledge that “we do know, for the 5 day rainfall event that the Clausius–Clapeyron relationship will hold” (Otto et al., 2023). Hence, these are well-known physical mechanisms (6). In this study two different event definitions to characterise the observed floods were analysed: the physics and future assessments provided additional lines of evidence to draw a strong conclusion for the 5 d event. They do not hold for the 60 d event that was also assessed. In this case the only line of evidence to draw from in the face of the highly uncertain results is the published literature (7). While not allowing for any quantitative conclusions, IPCC reports and individual studies did provide support for the conclusion that “climate change increased extreme monsoon rainfall” as the overarching headline result.
3.2 Overarching message
Following this structured assessment, there is of course a wider context to be taken into account before finalising the overarching message. The approach of extreme-event attribution taken here is one of different possible framings of attribution studies and, as has been discussed extensively, different framings lead to different attribution results (see e.g. Jézéquel et al., 2018; Stott et al., 2016; Otto et al., 2016; Chen et al., 2021). The same is true for different event definitions (Leach et al., 2020). Thus, there is always an element of choice of definition and framing in the overarching message, but in some cases this plays a smaller role than in others. For example, the definition for the rainfall associated with Hurricane Harvey (van Oldenborgh et al., 2017) is temporally and spatially very distinct, while the rainfall is also Gumbel-distributed, so that the probability ratio is independent of the return period and thus the magnitude of the event. In other cases however the event definition is much less clear, e.g. when impacts accumulate over several individual storms or no impacts are reported. Thus, confidence in the case of Harvey is higher than in the case of the Emilia-Romagna floods, despite similarly strong evidence.
Furthermore, the framing used in this approach tends to underestimate the role of climate change in data-sparse regions, as discussed e.g. in Otto et al. (2022), which means that focusing more on the upper bounds could be the more appropriate approach if e.g. the physical reasoning would support an increase, but the models do not show a significant change.
“What is the role of climate change?” This is a simple question climate scientists get asked constantly that very often does not have an easy answer. While there are many papers discussing the value of different methods of attribution, e.g. that of Jézéquel et al. (2018), there is a lack of methods beyond what we describe here to quantitatively combine observation and model-based results to reach overarching attribution statements. One exception is a Bayesian framework implemented by Robin and Ribes (2020) in which climate models are used to define prior distributions for the parameters of the extreme-value distribution and, combined with observations, to estimate the extent to which the event of interest has been affected by climate change. With meteorological services around the world starting to implement event attribution in operational frameworks, we hope that new methods to synthesise different data sources and other lines of evidence in addition to the one we have shown here will evolve to establish best practices and trustworthy methods to inform policy, e.g. in the context of loss and damage (Noy et al., 2023). The methods used here are chosen to be quick to implement so that they can be used in rapid or even automated attribution studies and be transparent in the weightings and assumptions that go into the synthesis routine. These methods were tested and improved in over 50 rapid attribution studies undertaken with the World Weather Attribution initiative and were found to be on the one hand standardised, which makes it easy to compare different studies with each other and keep a degree of methodological neutrality. On the other hand, they are flexible enough to allow different interpretations given very different contexts. The logical next step would be to implement a Bayesian framework following e.g. Robin and Ribes (2020) that would include some of the lines of evidence we now use on top of the statistical method to interpret the results and craft overarching messages to be used as a prior, e.g. physical understanding. Such a framing would also allow for more conditional methods of event attribution to be included in the same overarching framing (Shepherd, 2016). When developing these it would be important to investigate how results obtained in a Bayesian framework can be assessed alongside studies using the synthesis routine developed here together with other previous probabilistic attribution studies as Bayesian methods are more elegant but less transparent. Simple and transparent methods to understand the role of climate change across a large range of geographies and types of hazard become increasingly important, with the increasing realisation that a more comprehensive evidence base of the impacts of human-induced climate change is needed to inform international climate policy (Otto and Fabian, 2023).
Changes can be defined over different time intervals. For instance, due to a lack of observational or model data, changes can be defined relative to 1950 rather than 1900 or another estimate of “pre-industrial”. Similarly, for a model with a time slice, the difference between the past and present climates can be different from the current difference in GMST levels (or years) between pre-industrial and “now”. It is preferable to calculate all measures over the same interval. However, if this is not possible because too few data would be left, the first step is to convert the PR and ΔI to a common time interval (Y1,Y2) from the individual observational and model intervals . For this we take the largest time interval: . Assuming a linear dependence on the smoothed global mean temperature T(y), the change in intensity can then be adjusted as
where we assume that we can extrapolate with the trend α given by the change in intensity divided by the change in smoothed global mean temperature. The same expression holds for log (PR). The bounds on the uncertainty interval are similarly transformed. As levels of warming in models can be very different from the observed change of 1.2, an alternative and recently more widely used method is to fix the warming level rather than the time period. This is done by assuming that the logarithm of the risk ratio depends linearly on the global mean temperature, just like for the probabilities themselves. This is done by transforming the model Δ GMST by a factor f, e.g. transforming a model Δ GMST of 1.0 to the observed Δ GMST of 1.2, where f=1.2.
The algorithms have been implemented in the public KNMI Climate Explorer web application at https://gitlab.com/KNMI-OSS/climexp/climexp_numerical/-/blob/master/src/synthesis.f90?ref_type=heads (Kew, 2024), and an R package, including functions to carry out non-stationary model fitting and analysis, currently available at https://github.com/WorldWeatherAttribution/rwwa (Barnes, 2024).
FELO, GJvO and RV developed the original idea. GJvO wrote the original code. FELO prepared the manuscript with contributions from CB, SK, SP and RV. CB rewrote the code in R, and SK updated the code in KNMI Climate Explorer.
At least one of the (co-)authors is a member of the editorial board of Advances in Statistical Climatology, Meteorology and Oceanography. The peer-review process was guided by an independent editor, and the authors also have no other competing interests to declare.
Publisher’s note: Copernicus Publications remains neutral with regard to jurisdictional claims made in the text, published maps, institutional affiliations, or any other geographical representation in this paper. While Copernicus Publications makes every effort to include appropriate place names, the final responsibility lies with the authors.
This research has been supported by the EU Horizon 2020 framework programme H2020 Science with and for Society (grant no. 101003469).
This paper was edited by Seung-Ki Min and reviewed by two anonymous referees.
Abramowitz, G., Herger, N., Gutmann, E., Hammerling, D., Knutti, R., Leduc, M., Lorenz, R., Pincus, R., and Schmidt, G. A.: ESD Reviews: Model dependence in multi-model climate ensembles: weighting, sub-selection and out-of-sample testing, Earth Syst. Dynam., 10, 91–105, https://doi.org/10.5194/esd-10-91-2019, 2019. a
Barnes, C.: WorldWeatherAttribution rwwa, Github [code], https://github.com/WorldWeatherAttribution/rwwa, last access: 29 October 2024. a
Chen, D., Rojas, M., Samset, B., Cobb, K., Niang, A. D., Edwards, P., Emori, S., Faria, S., Hawkins, E., Hope, P., Huybrechts, P., Meinshausen, M., Mustafa, S., Plattner, G.-K., and Tréguier, A.-M.: Framing, Context, and Methods, in: Framing, Context, and Methods. In Climate Change 2021: The Physical Science Basis. Contribution of Working Group I to the Sixth Assessment Report of the Intergovernmental Panel on Climate Change, edited by: Masson-Delmotte, V. et al., chap. 1, 147–286, Cambridge University Press, Cambridge, U.K. and New York, U.S.A., https://doi.org/10.1017/9781009157896.003, 2021. a, b
Eyring, V., Gillett, N. P., Achuta Rao, K. M., Barimalala, R., Barreiro Parrillo, M., Bellouin, N., Cassou, C., Durack, P., Kosaka, Y., McGregor, S., Min, S., Morgenstern, O., and Sun, Y.: Human Influence on the Climate System, in: Human Influence on the Climate System. In Climate Change 2021: The Physical Science Basis. Contribution of Working Group I to the Sixth Assessment Report of the Intergovernmental Panel on Climate Change, edited by: Masson-Delmotte, V. et al., chap. 1, 423–552, Cambridge University Press, Cambridge, U.K. and New York, U.S.A., https://doi.org/10.1017/9781009157896.005, 2021. a
Haughton, N., Abramowitz, G., Pitman, A., and Phipps, S. J.: Weighting climate model ensembles for mean and variance estimates, Clim. Dynam., 45, 3169–3181, https://doi.org/10.1007/s00382-015-2531-3, 2015. a
Hauser, M., Gudmundsson, L., Orth, R., Jézéquel, A., Haustein, K., Vautard, R., van Oldenborgh, G. J., Wilcox, L., and Seneviratne, S. I.: Methods and Model Dependency of Extreme Event Attribution: The 2015 European Drought, Earth's Future, 5, 1034–1043, https://doi.org/10.1002/2017EF000612, 2017. a
Jézéquel, A., Dépoues, V., Guillemot, H., Trolliet, M., Vanderlinden, J.-P., and Yiou, P.: Behind the veil of extreme event attribution, Climatic Change, 149, 367–383, https://doi.org/10.1007/s10584-018-2252-9, 2018. a, b, c
Kew, S.: Climate Explorer Repository, Gitlab [code], https://gitlab.com/KNMI-OSS/climexp/climexp_numerical/-/blob/master/src/synthesis.f90?ref_type=heads, last access: 29 October 2024. a
Kew, S. F., Philip, S. Y., Hauser, M., Hobbins, M., Wanders, N., van Oldenborgh, G. J., van der Wiel, K., Veldkamp, T. I. E., Kimutai, J., Funk, C., and Otto, F. E. L.: Impact of precipitation and increasing temperatures on drought trends in eastern Africa, Earth Syst. Dynam., 12, 17–35, https://doi.org/10.5194/esd-12-17-2021, 2021. a, b
Leach, N. J., Li, S., Sparrow, S., van Oldenborgh, G. J., Lott, F. C., Weisheimer, A., and Allen, M. R.: Anthropogenic Influence on the 2018 Summer Warm Spell in Europe: The Impact of Different Spatio-Temporal Scales, B. Am. Meteorol. Soc., 101, S41–S46, https://doi.org/10.1175/BAMS-D-19-0201.1, 2020. a, b
Li, S. and Otto, F. E. L.: The role of human-induced climate change in heavy rainfall events such as the one associated with Typhoon Hagibis, Climatic Change, 172, 1573–1480, https://doi.org/10.1007/s10584-022-03344-9, 2022. a
Lloyd, E. A. and Oreskes, N.: Climate Change Attribution: When Is It Appropriate to Accept New Methods?, Earth's Future, 6, 311–325, https://doi.org/10.1002/2017EF000665, 2018. a, b
Merrifield, A. L., Brunner, L., Lorenz, R., Medhaug, I., and Knutti, R.: An investigation of weighting schemes suitable for incorporating large ensembles into multi-model ensembles, Earth Syst. Dynam., 11, 807–834, https://doi.org/10.5194/esd-11-807-2020, 2020. a
National Academies of Sciences, Engineering, and Medicine: Attribution of Extreme Weather Events in the Context of Climate Change, The National Academies Press, https://doi.org/10.17226/21852, 2016. a, b
Noy, I., Wehner, M., Stone, D., Rosier, S., Frame, D., Lawal, K. A., and Newman, R.: Event attribution is ready to inform loss and damage negotiations, Nat. Clim. Change, 13, 1279–1281, https://doi.org/10.1038/s41558-023-01865-4, 2023. a
Otto, F. E.: Attribution of Extreme Events to Climate Change, Annu. Rev. Env. Resour., 48, 813–828, https://doi.org/10.1146/annurev-environ-112621-083538, 2023. a
Otto, F. E. L. and Fabian, F.: Equalising the evidence base for adaptation and loss and damages, Glob. Policy, 15, 64–74, https://doi.org/10.1111/1758-5899.13269, 2023. a
Otto, F. E. L., van Oldenborgh, G. J., Eden, J. M., Stott, P. A., Karoly, D. J., and Allen, M. R.: The attribution question, Nat. Clim. Change, 6, 813–816, https://doi.org/10.1038/nclimate3089, 2016. a
Otto, F. E. L., Kew, S., Philip, S., Stott, P., and Oldenborgh, G. J. V.: How to Provide Useful Attribution Statements: Lessons Learned from Operationalizing Event Attribution in Europe, B. Am. Meteorol. Soc., 103, S21–S25, https://doi.org/10.1175/BAMS-D-21-0267.1, 2022. a
Otto, F. E. L., Zachariah, M., Saeed, F., Siddiqi, A., Kamil, S., Mushtaq, H., Arulalan, T., AchutaRao, K., Chaithra, S. T., Barnes, C., Philip, S., Kew, S., Vautard, R., Koren, G., Pinto, I., Wolski, P., Vahlberg, M., Singh, R., Arrighi, J., van Aalst, M., Thalheimer, L., Raju, E., Li, S., Yang, W., Harrington, L. J., and Clarke, B.: Climate change increased extreme monsoon rainfall, flooding highly vulnerable communities in Pakistan, Environmental Research: Climate, 2, 025001, https://doi.org/10.1088/2752-5295/acbfd5, 2023. a, b
Philip, S. Y., Kew, S. F., van Oldenborgh, G. J., Aalbers, E., Otto, F. E. L., Haustein, K., Habets, F., and Singh, R.: Validation of a rapid attribution of the May/June 2016 flood-inducing precipitation in France to climate change, J. Hydrometeorol., 19, 1881–1898, https://doi.org/10.1175/JHM-D-18-0074.1, 2018. a, b
Philip, S., Kew, S., van Oldenborgh, G. J., Otto, F., Vautard, R., van der Wiel, K., King, A., Lott, F., Arrighi, J., Singh, R., and van Aalst, M.: A protocol for probabilistic extreme event attribution analyses, Adv. Stat. Clim. Meteorol. Oceanogr., 6, 177–203, https://doi.org/10.5194/ascmo-6-177-2020, 2020. a, b, c, d, e
Robin, Y. and Ribes, A.: Nonstationary extreme value analysis for event attribution combining climate models and observations, Adv. Stat. Clim. Meteorol. Oceanogr., 6, 205–221, https://doi.org/10.5194/ascmo-6-205-2020, 2020. a, b
Sansom, P. G., Stephenson, D. B., Ferro, C. A. T., Zappa, G., and Shaffrey, L.: Simple Uncertainty Frameworks for Selecting Weighting Schemes and Interpreting Multimodel Ensemble Climate Change Experiments, J. Climate, 26, 4017–4037, https://doi.org/10.1175/JCLI-D-12-00462.1, 2013. a
Shepherd, T. G.: A Common Framework for Approaches to Extreme Event Attribution, Current Climate Change Reports, 2, 28–38, https://doi.org/10.1007/s40641-016-0033-y, 2016. a, b
Stocker, T. F., Sin, D., Plattner, G.-K., Tignor, M. M. B., Allen, S. K., Boschung, J., Nauels, A., Xia, Y., Bex, V., and Midgley, P. M. (Eds.): Climate Change 2013: The Physical Science Basis, Cambridge University Press, Cambridge, U.K. and New York, U.S.A., ISBN-13 978-1107057999, 2013. a
Stott, P. A., Christidis, N., Otto, F. E. L., Sun, Y., Vanderlinden, J.-P., van Oldenborgh, G. J., Vautard, R., von Storch, H., Walton, P., Yiou, P., and Zwiers, F. W.: Attribution of extreme weather and climate-related events, WIREs Clim. Change, 7, 23–41, https://doi.org/10.1002/wcc.380, 2016. a, b
van Oldenborgh, G. J., van der Wiel, K., Sebastian, A., Singh, R. K., Arrighi, J., Otto, F. E. L., Haustein, K., Li, S., Vecchi, G. A., and Cullen, H.: Attribution of extreme rainfall from Hurricane Harvey, August 2017, Environ. Res. Lett., 12, 124009, https://doi.org/10.1088/1748-9326/aa9ef2, 2017. a
van Oldenborgh, G. J., Philip, S., Kew, S., van Weele, M., Uhe, P., Otto, F., Singh, R., Pai, I., Cullen, H., and AchutaRao, K.: Extreme heat in India and anthropogenic climate change, Nat. Hazards Earth Syst. Sci., 18, 365–381, https://doi.org/10.5194/nhess-18-365-2018, 2018. a
van Oldenborgh, G. J., Otto, F. E. L., Vautard, R., van der Wiel, K., Kew, S. F., Philip, S. Y., King, A. D., Lott, F. C., Arrighi, J., Singh, R., and van Aalst, M. K.: Pathways and pitfalls in extreme event attribution, Climatic Change, 166, 13, https://doi.org/10.1007/s10584-021-03071-7, 2021. a, b
van Oldenborgh, G. J., Vautard, R., Otto, F. E. L., Seneviratne, S. I., Wehner, M. F., Stott, P. A., Hegerl, G. C., Philip, S. Y., and Kew, S. F.: Attributing and projecting heatwaves is hard: we can do better, Earth's Future, 10, e2021EF002271, https://doi.org/10.1029/2021EF002271, 2022. a
Vautard, R., Yiou, P., Otto, F., Stott, P., Christidis, N., van Oldenborgh, G. J., and Schaller, N.: Attribution of human-induced dynamical and thermodynamical contributions in extreme weather events, Environ. Res. Lett., 11, 114009, https://doi.org/10.1088/1748-9326/11/11/114009, 2016. a
Vautard, R., van Oldenborgh, G. J., Otto, F. E. L., Yiou, P., de Vries, H., van Meijgaard, E., Stepek, A., Soubeyroux, J.-M., Philip, S., Kew, S. F., Costella, C., Singh, R., and Tebaldi, C.: Human influence on European winter wind storms such as those of January 2018, Earth Syst. Dynam., 10, 271–286, https://doi.org/10.5194/esd-10-271-2019, 2019. a, b, c
Zeder, J., Sippel, S., Pasche, O. C., Engelke, S., and Fischer, E. M.: The Effect of a Short Observational Record on the Statistics of Temperature Extremes, Geophys. Res. Lett., 50, e2023GL104090, https://doi.org/10.1029/2023GL104090, 2023. a
This is typically true except in regions where the weather is strongly influenced by slowly varying boundary conditions such as sea surface temperatures, in which case noticeably different model behaviour would be observed for models forced with SSTs compared to coupled models.